This is a Preprint and has not been peer reviewed. The published version of this Preprint is available: https://doi.org/10.1111/2041-210X.14200. This is version 4 of this Preprint.
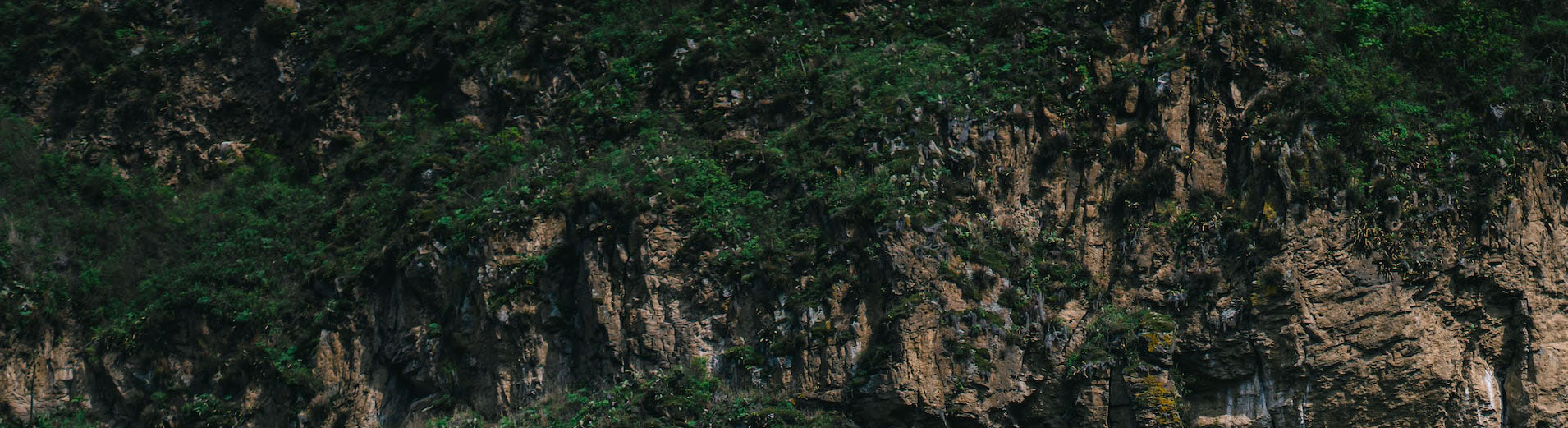
Downloads
Authors
Abstract
1. Assessing the biological relevance of variance components estimated using MCMC-based mixed-effects models is not straightforward. Variance estimates are constrained to be greater than zero and their posterior distributions are often asymmetric. Different measures of central tendency for these distributions can therefore vary widely, and credible intervals cannot overlap zero, making it difficult to assess the size and statistical support for among-group variance. Statistical support is often assessed through visual inspection of the whole posterior distribution and so relies on subjective decisions for interpretation.
2. We use simulations to demonstrate the difficulties of summarising the posterior distributions of variance estimates from MCMC-based models. We then describe different methods for generating the expected null distribution (i.e. a distribution of effect sizes that would be obtained if there was no among-group variance) that can be used to aid in the interpretation of variance estimates.
3. Through comparing commonly used summary statistics of posterior distributions of variance components, we show that the posterior median is predominantly the least biased. We further show how null distributions can be used to derive a p-value that provides complementary information to the commonly presented measures of central tendency and uncertainty. Finally, we show how these p-values facilitate the implementation of power analyses within an MCMC framework.
4. The use of null distributions for variance components can aid study design and the interpretation of results from MCMC-based models. We hope that this manuscript will make empiricists using mixed models think more carefully about their results, what descriptive statistics they present and what inference they can make.
DOI
https://doi.org/10.32942/X27G6W
Subjects
Ecology and Evolutionary Biology, Life Sciences
Keywords
hierarchical modelsvariance, null distribution, permutation, simulations, squidSim, Hierarchical Models, variance
Dates
Published: 2022-11-27 08:39
Last Updated: 2023-07-22 03:17
Older Versions
License
CC-BY Attribution-NonCommercial 4.0 International
Additional Metadata
Conflict of interest statement:
None
Data and Code Availability Statement:
All code and generated data for the simulated examples are available at https://github.com/squidgroup/null_distributions
There are no comments or no comments have been made public for this article.