This is a Preprint and has not been peer reviewed. This is version 1 of this Preprint.
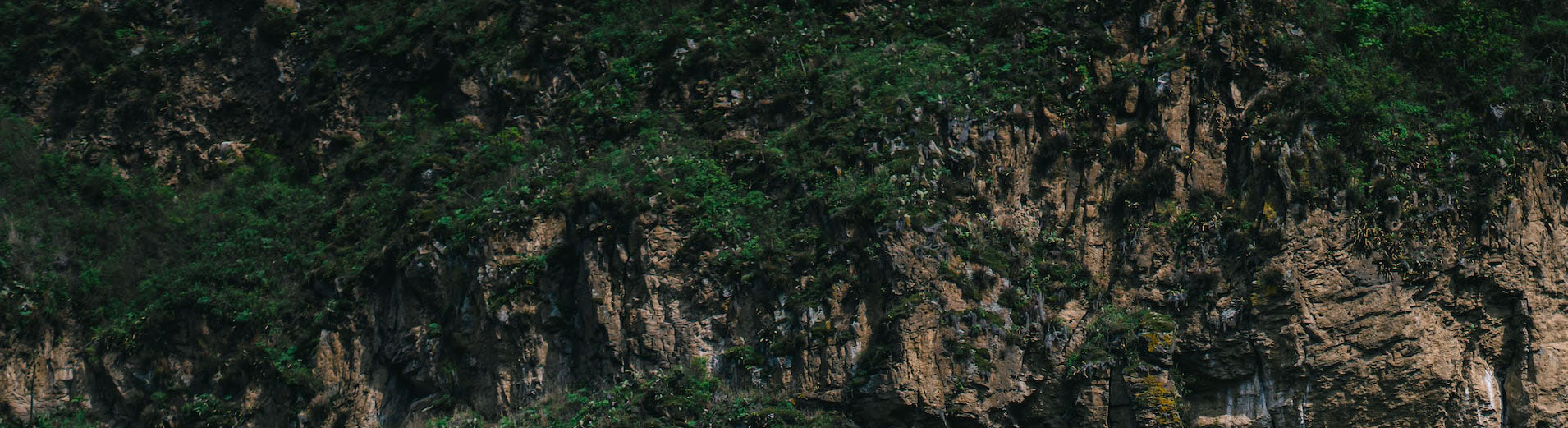
Downloads
Authors
Abstract
1. Biodiversity monitoring schemes periodically measure species’ abundances and distributions at a sample of sites to understand how they have changed over time. Often, the aim is to infer change in an average sense across some wider landscape. Inference to the wider landscape is simple if the species’ abundances and distributions are similar at sampled to non-sampled locations. Otherwise, the data are geographically biased, and some form of correction is desirable.
2. We combine causal diagrams with “superpopulation models” to correct time-varying geographic biases in biodiversity monitoring data. For a given time-period, expert-derived causal diagrams are used to deduce the set of variables that explain the geographic bias, and superpopulation models adjust for these variables to produce a corrected estimate of a landscape-wide mean of e.g. abundance or occupancy. Estimating a time trend in the variable of interest is achieved by fitting models for multiple time-periods and, if the drivers of bias are suspect to change over time, by constructing per period causal diagrams. We test the approach using simulated data then apply it to real data from the UK Butterfly Monitoring Scheme (UKBMS).
3. Where the variables that explain the geographic bias are known and measured without error, our method is unbiased. Introducing measurement error reduces the method’s efficacy, but it is still an improvement on using the sample mean. When applied to data from the UKBMS, the approach gives different results to the scheme’s current method, which assumes no geographic bias.
4. Where the goal is to estimate change in some variable of interest at the landscape level (e.g. biodiversity indicators), models that do not adjust for geographic bias implicitly assume it does not exist. Our approach makes the weaker assumption that there is no geographic bias conditional on the adjustment variables, so it should yield more accurate estimates of time trends in many circumstances. The method does require assumptions about the drivers of bias, but these are codified explicitly in the causal diagrams. Operationalising our approach should be less costly than full probability sampling, which would be needed to satisfy the assumptions of conventional approaches.
DOI
https://doi.org/10.32942/X2991W
Subjects
Life Sciences
Keywords
Directed acyclic graph, expert consultation, imputation, sampling bias, species abundance, time trend
Dates
Published: 2024-04-18 22:18
Last Updated: 2024-04-19 02:18
License
CC BY Attribution 4.0 International
Additional Metadata
Language:
English
Conflict of interest statement:
None to declare.
Data and Code Availability Statement:
The butterfly data must be requested from the UK Butterfly Monitoring Scheme at https://ukbms.org/request-data. All code used to conduct our analyses are provided in the supplementary materials. We will deposit the code on Zenodo should the article be accepted for publication.
There are no comments or no comments have been made public for this article.