This is a Preprint and has not been peer reviewed. The published version of this Preprint is available: https://doi.org/10.1093/iob/obae036. This is version 4 of this Preprint.
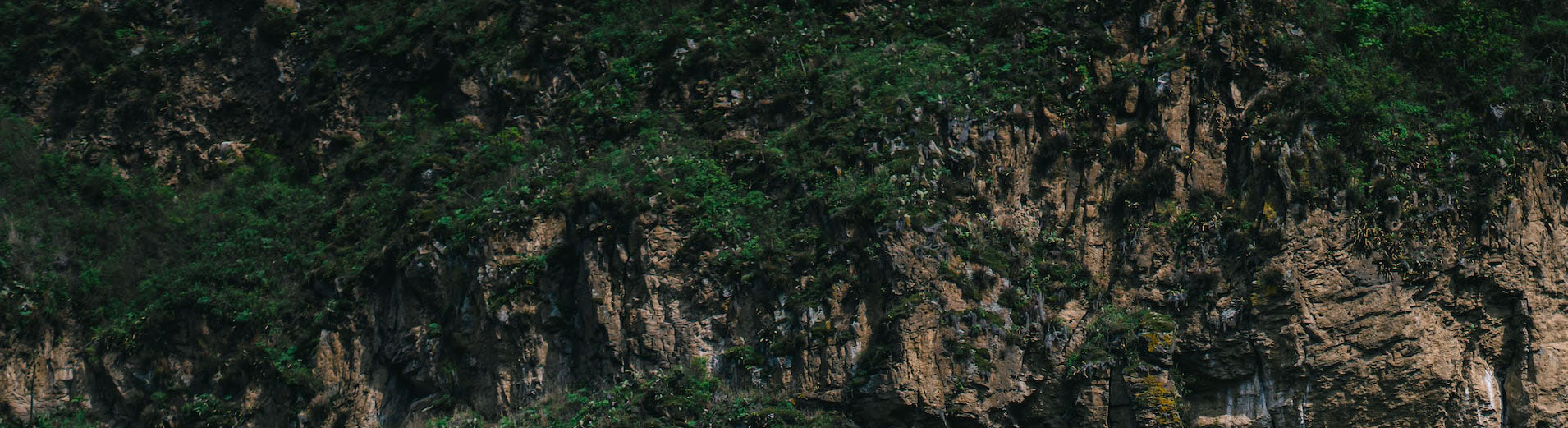
Downloads
Authors
Abstract
Artificial intelligence (AI) is poised to revolutionise many aspects of science, including the study of evolutionary morphology. While classical AI methods such as principal component analysis and cluster analysis have been commonplace in the study of evolutionary morphology for decades, recent years have seen increasing application of deep learning to ecology and evolutionary biology. As digitised specimen databases become increasingly prevalent and openly available, AI is offering vast new potential to circumvent long standing barriers to rapid, big data analysis of phenotypes. Here, we review the current state of AI methods available for the study of evolutionary morphology, which are most developed in the area of data acquisition and processing. We introduce the main available AI techniques, categorising them into three stages based on their order of appearance: (i) machine learning, (ii) deep learning, and (iii) the most recent advancements in large-scale models and multimodal learning. Next, we present case studies of existing approaches using AI for evolutionary morphology, including image capture and segmentation, feature recognition, morphometrics, and phylogenetics. We then discuss the prospectus for near-term advances in specific areas of inquiry within this field, including the potential of new AI methods that have not yet been applied to the study of morphological evolution. In particular, we note key areas where AI remains underutilised and could be used to enhance studies of evolutionary morphology. This combination of current methods and potential developments have the capacity to transform the evolutionary analysis of the organismal phenotype into evolutionary phenomics, leading to an era of “Big Data'' that aligns the study of phenotypes with genomics and other areas of bioinformatics.
DOI
https://doi.org/10.32942/X2S315
Subjects
Life Sciences
Keywords
Artificial Intelligence, Evolutionary morphology, Phenomics, machine learning
Dates
Published: 2024-01-17 06:09
Last Updated: 2024-10-02 21:51
Older Versions
License
CC-BY Attribution-NonCommercial 4.0 International
Additional Metadata
Language:
English
Conflict of interest statement:
None
Data and Code Availability Statement:
No new data were generated or analysed in support of this research. The tools table in this paper will be kept updated at [phenomeAI.org].
There are no comments or no comments have been made public for this article.