This is a Preprint and has not been peer reviewed. The published version of this Preprint is available: https://doi.org/10.24072/pci.ecology.100640. This is version 4 of this Preprint.
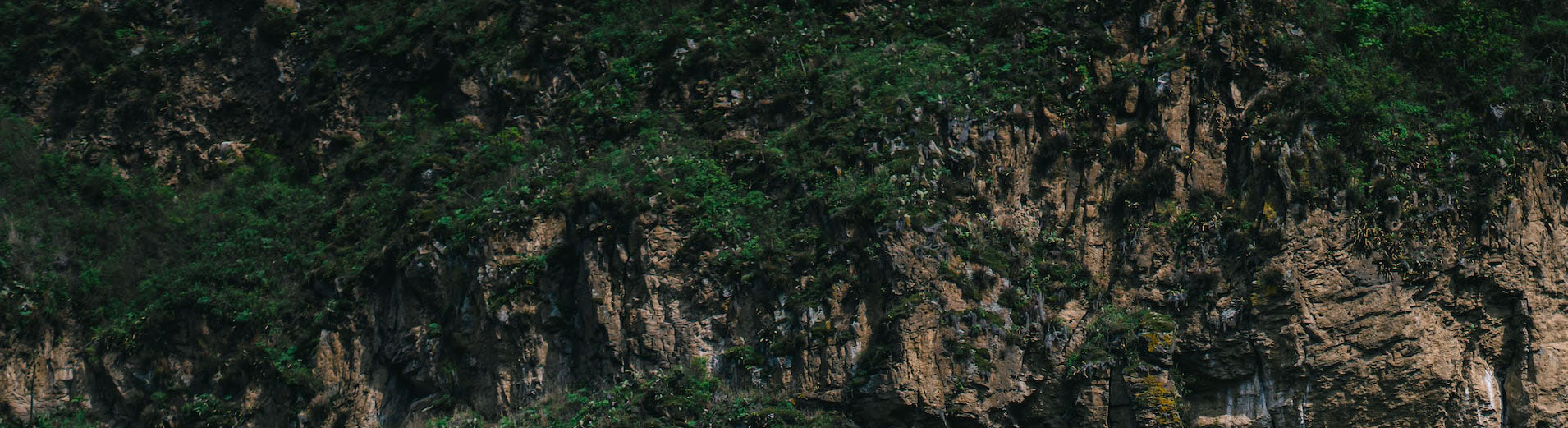
Using multiple datasets to account for misalignment between statistical and biological populations for abundance estimation
Downloads
Authors
Abstract
A fundamental aspect of ecology is identifying and characterizing population processes. Because a complete census is rare, we almost always use sampling to make inference about the biological population, and the part of the population at risk of sampling is referred to as the statistical population. Ideally, the statistical population is the same as, or accurately represents its corresponding biological population. However, in practice, they rarely align in space and time, which can lead to biased inference. We often view a population misalignment as a temporary emigration process and resolve it with replicate and/or repeat sampling, though this approach is not feasible for all species and habitats. We developed a hierarchical modeling framework to estimate abundance of a biological population of the Kittlitz’s murrelet (Brachyramphus brevirostris), a highly mobile, non-territorial, ice-associated seabird of conservation concern in Alaska and eastern Russia. Our framework combines datasets from boat and telemetry surveys to account for all components of detection probability, specifically using telemetry locations to estimate probability of presence (pp) and line transect distance sampling to estimate probability of detection (pd). By estimating pp directly, we were able to account for temporary emigration from the sampled area, which changed with shifting icefloes between sampling occasions. Between 2007 and 2012, annual pp was highly variable, ranging from 0.33 to 0.75 (median=0.50, standard deviation=0.02), but was not predictable using five environmental covariates. In years when two boat surveys were conducted, our model reduced the coefficient of variation (CV) of abundance estimates for the biological population compared to the statistical population by 13–35%, yet in the year with only one boat survey (2009), the CV skyrocketed about 10-fold, emphasizing the importance of a second survey if pp varies. Although we increased the precision of annual abundance estimates by accounting for pp, we did not see the same improvement in the temporal trend estimate. This result indicates that while we reduced within-year variance, we failed to account for a source(s) of variation across years, which we suspect is related to the propensity for murrelets to skip breeding in some years. Our modeling framework to account for a population misalignment is simple, flexible, and scalable for generating unbiased and precise abundance estimates of highly mobile species that occupy dynamic habitats where other open population models are not possible. Importantly, it improves inference of the biological population, which is the population of interest. We urge ecologists to think critically about the population in which they want to draw inference, especially as tracking technology improves and model complexity increases.
DOI
https://doi.org/10.32942/X2W03T
Subjects
Life Sciences
Keywords
temporary emigration, biological population, statistical population, integrated model, Detection probability, seabird, Brachyramphus, superpopulation, Brachyramphus murrelet
Dates
Published: 2023-12-28 08:00
Last Updated: 2024-11-11 18:52
Older Versions
License
CC BY Attribution 4.0 International
Additional Metadata
Conflict of interest statement:
The authors declare no conflict of interest.
Data and Code Availability Statement:
All data collected between 2005 and 2012 that were used in this manuscript are available via Dryad at https://doi.org/10.5061/dryad.0cfxpnw8m. However, boat survey data from 2016 and 2017 were collected by the Alaska Department of Fish and Game, who considers these data to be sensitive and has withheld them in accordance with Alaska State Statute 16.05.815(d). Request of these data can be made to: Wildlife Science Director, Alaska Department of Fish and Game, Division of Wildlife Conservation, 1255 West 8th St., Juneau, Alaska, 99802 or to dfg.dwc.director@alaska.gov.
Language:
English
There are no comments or no comments have been made public for this article.