This is a Preprint and has not been peer reviewed. This is version 1 of this Preprint.
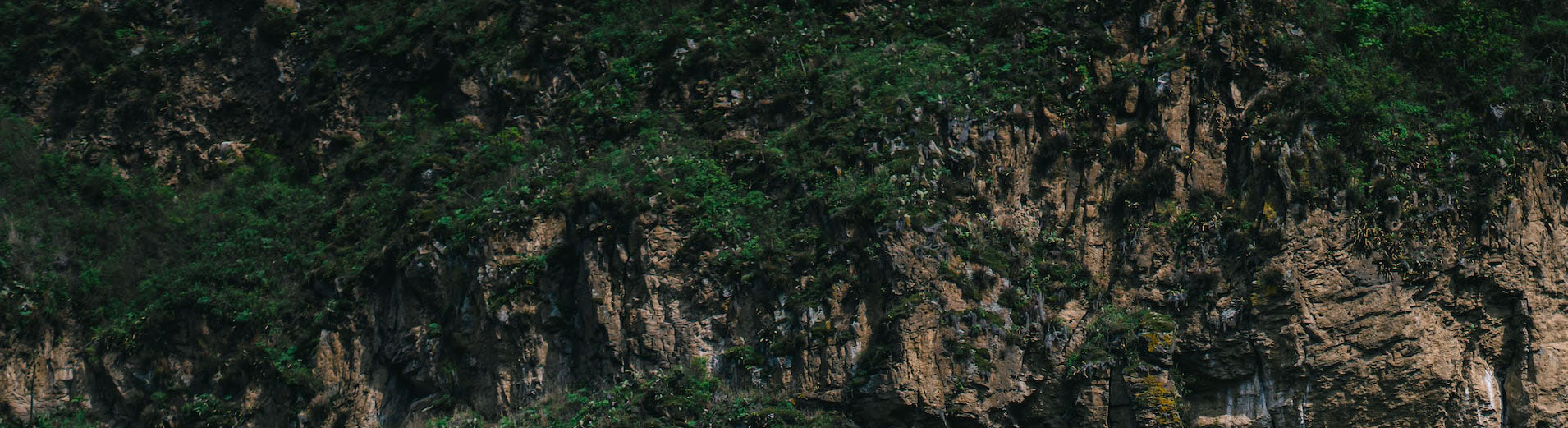
Downloads
Authors
Abstract
Species distribution models (SDMs) have proven valuable in filling gaps in our knowledge of species occurrences. However, despite their broad applicability, SDMs exhibit critical shortcomings due to limitations in species occurrence data. These limitations include, in particular, issues related to sample size, positional error, and sampling bias. In addition, it is widely recognized that the quality of SDMs as well as the approaches used to mitigate the impact of the aforementioned data limitations are dependent on species ecology. While numerous studies have experimentally evaluated the effects of these data limitations on SDM performance, a synthesis of their results is lacking. However, without a comprehensive understanding of their individual and combined effects, our ability to predict the influence of these issues on the quality of modelled species-environment associations remains largely uncertain, limiting the value of model outputs. In this paper, we review studies that have evaluated the effects of sample size, positional error, sampling bias, and species ecology on SDMs outputs. We integrate their findings into a step-by-step guide for critical assessment of spatial data intended for use in SDMs.
DOI
https://doi.org/10.32942/X2JS5D
Subjects
Life Sciences
Keywords
EcolEcogical Niche Modelling, Validation, Quality, training, Scale, Sampling, heterogeneity, Filtering, Ecological Niche Modelling, complexity
Dates
Published: 2023-12-04 07:23
Last Updated: 2023-12-04 07:23
License
CC BY Attribution 4.0 International
Additional Metadata
Language:
English
Comment #133 Oliver Pescott @ 2023-12-05 08:09
Shouldn't you include Boyd et al. (2023) in Table 3? Those authors used standard approaches to modelling nonprobability samples within the SDM world (i.e. standard statistical models and approaches to pseudo-absence placement), and evaluated ~500 models via expert feedback, thus partly overcoming issues with in-sample-only measures of model performance (at least as far as we can accept that taxon-group experts have some idea of the environmental determinants of species' distributions and experience of their realised niches). It seems to me that Boyd et al. (2023) warrants considerably more discussion in your manuscript than is currently the case (full disclosure: yes, I am the senior author on that paper).