This is a Preprint and has not been peer reviewed. This is version 9 of this Preprint.
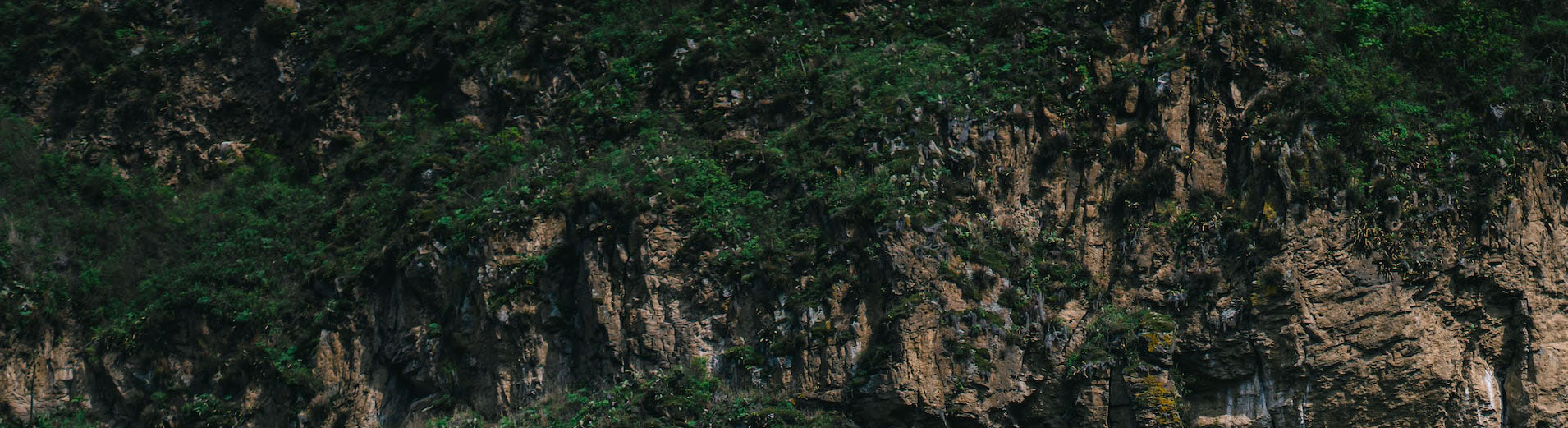
Downloads
Supplementary Files
Authors
Abstract
Spatial patterns in population trends, particularly those at finer geographic scales, can help us better understand the factors driving population change in North American birds. The standard trend models for the North American Breeding Bird Survey (BBS) were designed to estimate trends within broad geographic strata, such as countries, Bird Conservation Regions, U.S. states, and Canadian territories or provinces. Calculating trend estimates at the level of the BBS’s individual survey transects (“routes”) allows us to explore finer spatial patterns and simultaneously estimate the effects of covariates, such as habitat loss or annual weather, on both relative abundance and trend (changes in relative abundance through time). Here, we describe four related hierarchical Bayesian models that estimate trends for individual BBS routes, implemented in the probabilistic programming language Stan. All four models estimate route-level trends and relative abundances using a hierarchical structure that shares information among routes, and three of the models share information in a spatially explicit way. The spatial models use either an intrinsic Conditional Autoregressive (iCAR) structure or a distance-based Gaussian Process (GP) to estimate the spatial components. We fit all four models to data for 71 species and then, because of the intensive computations required, fit two of the models (one spatial and one non-spatial) for an additional 216 species. In a leave-future-out cross-validation, the spatial models outperformed the non-spatial models for 284 out of 287 species. The best approach to modeling the spatial components depends on the species being modeled; the Gaussian Process had the highest predictive accuracy for 69% of the species tested here and the iCAR was better for the remaining 31%. We also present two examples of route-level covariate analyses focused on spatial and temporal variation in habitat for Rufous Hummingbird (Selasphorus rufus) and Horned Grebe (Podiceps auritus). In both examples, the inclusion of covariates improved our understanding of the patterns in the rate of population change for both species. Route-level models for BBS data are useful for visualizing spatial patterns of population change, generating hypotheses on the causes of change, comparing patterns of change among regions and species, and testing hypotheses on causes of change with relevant covariates.
DOI
https://doi.org/10.32942/X2SP5J
Subjects
Biodiversity, Biostatistics, Ecology and Evolutionary Biology, Environmental Monitoring, Population Biology, Statistical Methodology, Statistical Models
Keywords
ecological monitoring, Gaussian Process, iCAR, population abundance, Gaussian Process, iCAR, Population Abundance, Population Trend
Dates
Published: 2023-10-27 19:51
Last Updated: 2024-01-23 01:55
Older Versions
- Version 8 - 2024-01-23
- Version 7 - 2024-01-23
- Version 6 - 2024-01-23
- Version 5 - 2024-01-23
- Version 4 - 2024-01-23
- Version 3 - 2024-01-23
- Version 2 - 2023-10-27
- Version 1 - 2023-10-27
License
CC BY Attribution 4.0 International
Additional Metadata
Language:
English
Conflict of interest statement:
None
Data and Code Availability Statement:
https://github.com/AdamCSmithCWS/Route-level_BBS_trends
There are no comments or no comments have been made public for this article.