This is a Preprint and has not been peer reviewed. The published version of this Preprint is available: https://doi.org/10.1016/j.ympev.2024.108066. This is version 2 of this Preprint.
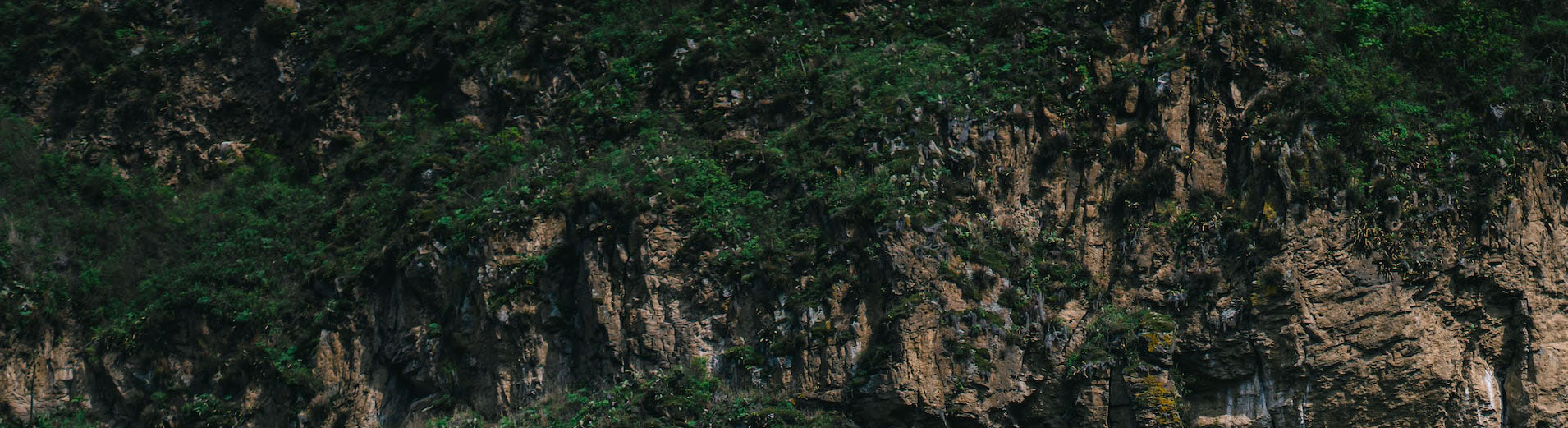
Applications of Machine Learning in Phylogenetics
Downloads
Authors
Abstract
Machine learning has increasingly been applied to a wide range of questions in phylogenetic
inference. Supervised machine learning approaches that rely on simulated training data have been
used to infer tree topologies and branch lengths, to select substitution models, and to perform
downstream inferences of introgression and diversification. Here, we review how researchers have
used several promising machine learning approaches to make phylogenetic inferences. Despite
the promise of these methods, several barriers prevent supervised machine learning from reaching
its full potential in phylogenetics. We discuss these barriers and potential paths forward. In the
future, we expect that the application of careful network designs and data encodings will allow
supervised machine learning to accommodate the complex processes that continue to confound
traditional phylogenetic methods.
DOI
https://doi.org/10.32942/X2XG7G
Subjects
Biology, Life Sciences
Keywords
Dates
Published: 2023-10-14 16:07
Last Updated: 2024-02-14 01:00
Older Versions
License
CC-By Attribution-NonCommercial-NoDerivatives 4.0 International
Additional Metadata
Language:
English
There are no comments or no comments have been made public for this article.