This is a Preprint and has not been peer reviewed. This is version 1 of this Preprint.
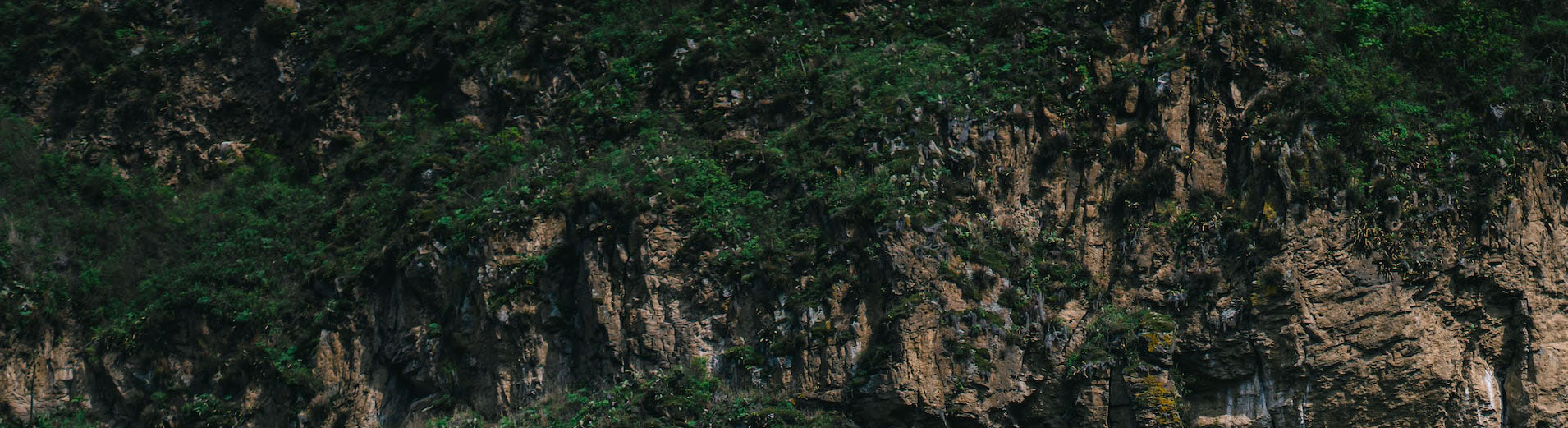
Downloads
Authors
Abstract
Leaf size varies within and between species, and previous work has linked this variation to the environment and evolutionary history separately. However, many previous studies fail to interlink both factors and are often data limited. To address this, our study developed a new workflow using machine learning to automate the extraction of leaf traits (leaf area, largest in-circle area and leaf curvature) from herbarium collections of Australian eucalypts (Eucalyptus, Angophora and Corymbia). Our dataset included 136,599 measurements, expanding existing data on this taxon’s leaf area by roughly 50-fold. With this dataset, we were able to confirm global positive relationships between leaf area and mean annual temperature and precipitation. Furthermore, we linked this trait-climate relationship to phylogeny, revealing large variation at the within-species level, potentially due to gene flow suppressing local adaptation. At deeper phylogenetic levels, the relationship strengthens and the slope converges towards the overall eucalypt slope, suggesting that the effect of gene flow relaxes just above the species level. The strengthening of trait-climate correlations just beyond the intraspecific level may represent a widespread phenomenon across various traits and taxa. Future studies may unveil these relationships with the larger sample sizes of new trait datasets generated through machine learning.
DOI
https://doi.org/10.32942/X2DG6F
Subjects
Life Sciences
Keywords
Artificial Intelligence, machine learning, climate, Trait Ecology, Leaf Traits, Leaf Area, Deep learning, computer vision
Dates
Published: 2023-05-05 15:46
License
CC BY Attribution 4.0 International
Additional Metadata
Language:
English
Conflict of interest statement:
None
There are no comments or no comments have been made public for this article.