This is a Preprint and has not been peer reviewed. This is version 3 of this Preprint.
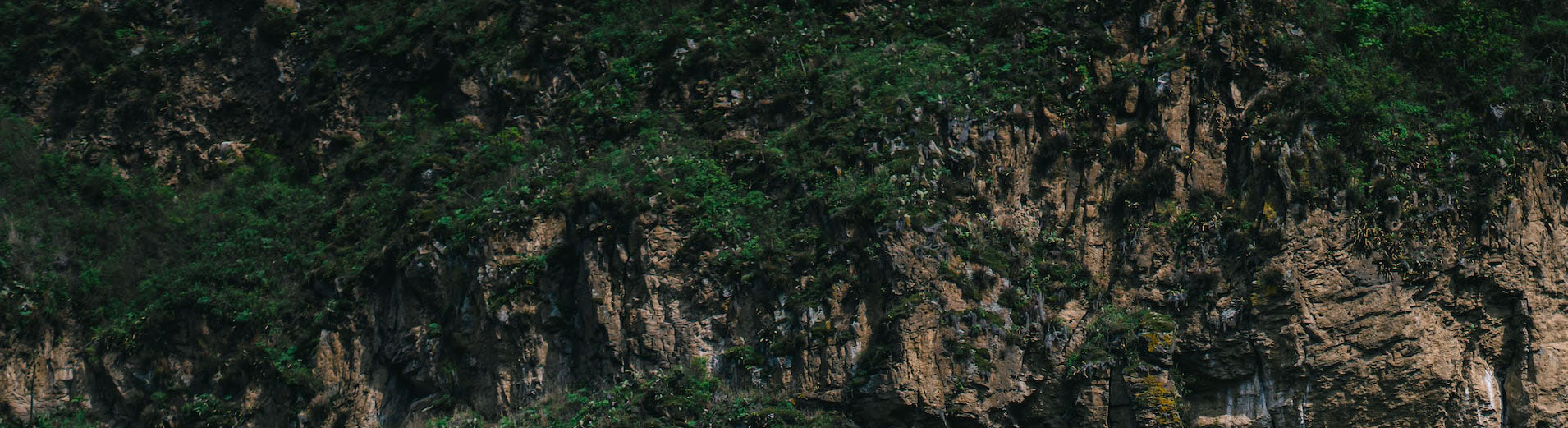
Downloads
Supplementary Files
Authors
Abstract
Forecasts of community dynamics are essential for the management of biodiversity. Theory suggests these predictions can be improved by leveraging multi-species dependencies to improve models, but empirical support for this is lacking. We test whether models that learn from multiple species, both to estimate nonlinear environmental effects and temporal dependence, improve forecasts for a semi-arid rodent community. Using Dynamic Generalized Additive Models, we analyze monthly captures for nine rodents over 25 years. We find strong evidence that multi-species dependencies improve performance, as models that captured these effects gave superior predictions. These models also provide novel insights, in our case by quantifying how changes in abundance for some species can have delayed, nonlinear effects on others while also uncovering important lagged effects of environmental drivers. We show that multivariate models are useful not only to improve ecological forecasts but also to ask targeted questions about community dynamics.
DOI
https://doi.org/10.32942/X2TS34
Subjects
Desert Ecology, Ecology and Evolutionary Biology, Life Sciences, Multivariate Analysis, Population Biology, Statistical Methodology, Statistical Models, Statistics and Probability
Keywords
ecological forecasting, Generalized additive model, population dynamics, regime shift, time series, vector autoregression
Dates
Published: 2023-03-13 14:26
Last Updated: 2024-03-11 15:33
Older Versions
License
CC BY Attribution 4.0 International
Additional Metadata
Data and Code Availability Statement:
R code to replicate data extraction / analyses is included in supplementary materials and will be uploaded to Zenodo on acceptance
There are no comments or no comments have been made public for this article.