This is a Preprint and has not been peer reviewed. The published version of this Preprint is available: https://doi.org/10.1111/geb.13793. This is version 3 of this Preprint.
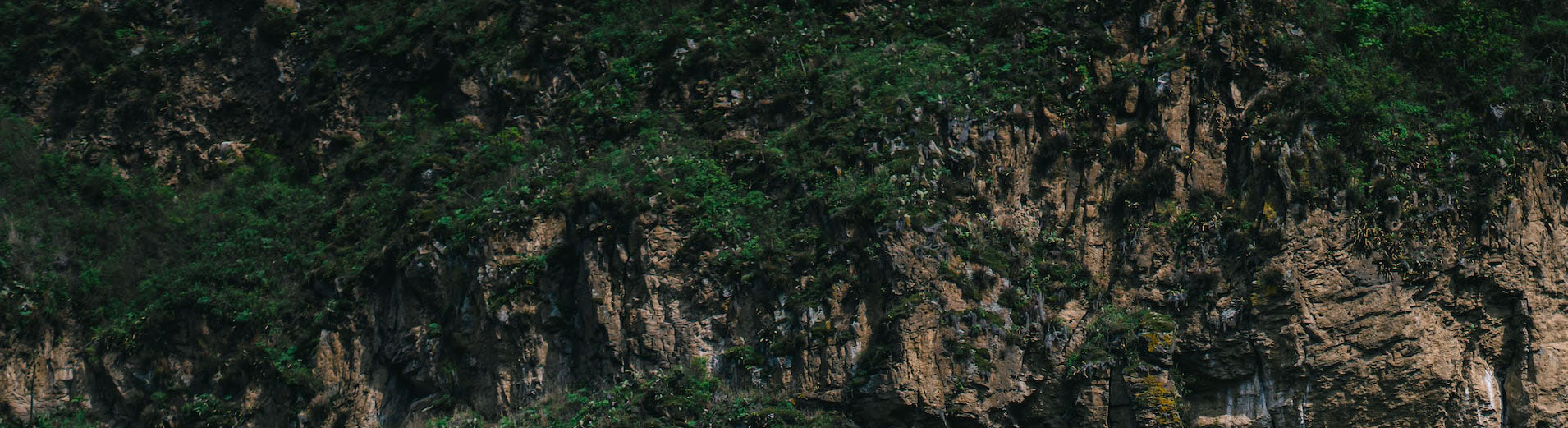
Quantifying uncertainties of ecological forecasting under climate change
Downloads
Authors
Abstract
Ecological forecasting is critical in understanding of ecological responses to climate change and is increasingly used in climate mitigation plans. The forecasts from correlative models can be challenged by novel environmental conditions and predictor collinearity that are common during model extrapolation. However, there is still a lack of comprehensive knowledge about how these factors interactively affect forecasting. We conducted modeling experiments to mimic a wide range of extrapolation scenarios commonly seen under climate change. We modeled three functional relationships using general linear model (GLM) and Random Forests algorithm (RF). We assessed how predictor novelty, training collinearity, collinearity shift, and model complexity interactively influenced model predictions. We found that predictor novelty and collinearity shift were two major factors for inflated errors. The prediction errors were doubled with moderate predictor novelty (predictors increased by 0.4) or considerable collinearity shift (correlation coefficient r changed > 0.9). Interestingly, we also found negative interactions between predictor novelty and collinearity shift. Model predictions became more erroneous as model complexity increased. Calibrating models using variables correlated with |r| < 0.7 has been a rule-of-thumb; building upon that, our study further recommends a threshold of < 0.4 increased predictor novelty and/or < 0.9 collinearity shift for forecasting models to avoid a substantial loss of model performance. GLM is a safer option than RF during forecasting because it is more tolerant to predictor novelty and collinearity shift when true predictors are known. One will be cautious with forecasting beyond our identified threshold regardless of modeling algorithm.
DOI
https://doi.org/10.32942/X2MS38
Subjects
Life Sciences
Keywords
climate changepredictor novelty, collinearity, collinearity shift, ecological forecasting, extrapolation, climate change, predictor novelty, Collinearity, collinearity shift, ecological forecasting, Extrapolation
Dates
Published: 2022-11-22 19:11
Last Updated: 2024-06-19 14:11
Older Versions
License
CC-BY Attribution-NonCommercial 4.0 International
Additional Metadata
Data and Code Availability Statement:
https://github.com/xinxxxin/Quantifying-uncertainties-of-ecological-forecasting-under-climate-change
There are no comments or no comments have been made public for this article.