This is a Preprint and has not been peer reviewed. The published version of this Preprint is available: https://doi.org/10.24072/pcjournal.261. This is version 4 of this Preprint.
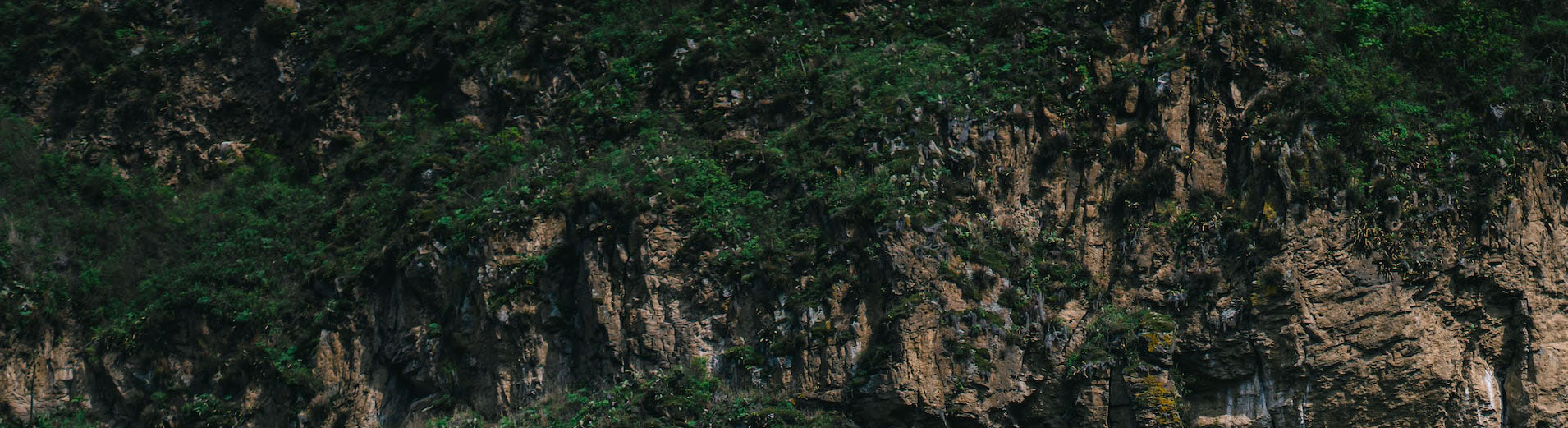
Rapid literature mapping on the recent use of machine learning for wildlife imagery
Downloads
Supplementary Files
Authors
Abstract
1. Machine (especially deep) learning algorithms are changing the way wildlife imagery is processed. They dramatically speed up the time to detect, count, classify animals and their behaviours. Yet, we currently have a very few systematic literature surveys on its use in wildlife imagery.
2. Through a literature survey (a ‘rapid’ review) and bibliometric mapping, we explored its use across: 1) species (vertebrates), 2) image types (e.g., camera traps, or drones), 3) study locations, 4) alternative machine learning algorithms, 5) outcomes (e.g., recognition, classification, or tracking), 6) reporting quality and openness, 7) author affiliation, and 8) publication journal types.
3. We found that increasing number of studies used convolutional neural networks (i.e., deep learning). Typically, studies have focused on large charismatic or iconic mammalian species . Increasing number of studies is published in ecology-specific journals indicating the uptake of deep learning to transform detection, classification and tracking of wildlife. Sharing of code was limited, with only 20% of studies providing links to analysis code.
4. Much of the published research and focus on animals came from India, China, Australia, or the USA. There were relatively few collaborations across countries. Given the power of machine learning, we recommend increasing collaboration and sharing approaches to utilise increasing amounts of wildlife imagery more rapidly and transform and improve understanding of wildlife behaviour and conservation.
5. Our survey augmented with bibliometric analyses provide valuable signposts for future studies to resolve and address shortcomings, gaps, and biases.
DOI
https://doi.org/10.32942/X2H59D
Subjects
Life Sciences
Keywords
machine learning, wildlife, Deep learning, computer vision, rapid review
Dates
Published: 2022-10-31 01:51
Last Updated: 2023-01-06 17:43
Older Versions
License
CC BY Attribution 4.0 International
Additional Metadata
Conflict of interest statement:
none
Data and Code Availability Statement:
Yes, it is all avaiable on a Github repository
There are no comments or no comments have been made public for this article.