This is a Preprint and has not been peer reviewed. This is version 1 of this Preprint.
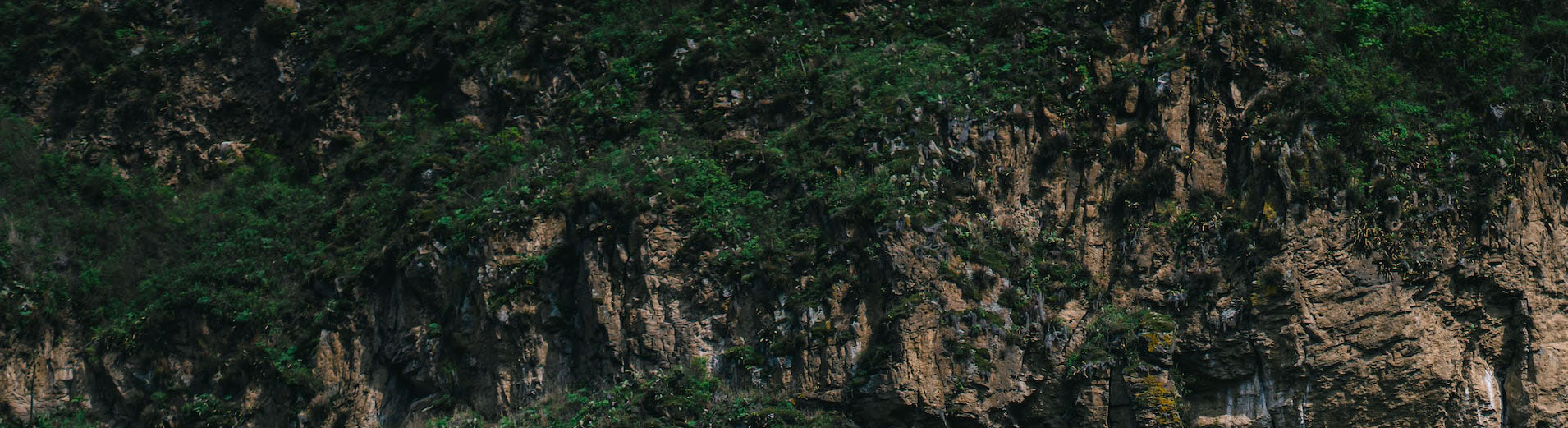
Learning from your mistakes: a novel method to predict the response to directional selection
Downloads
Authors
Abstract
Predicting how populations respond to selection is a key goal of evolutionary biology. The field of quantitative genetics provides predictions for the response to directional selection through the breeder’s equation. However, differences between the observed responses to selection and those predicted by the breeder’s equation occur. The sources of these errors include omission of traits under selection, inaccurate estimates of genetic variance, and nonlinearities in the relationship between genetic and phenotypic variation. A key insight from previous research is that the expected value of these prediction errors is often not zero, in which case the predictions are systematically biased. Here, we propose that this prediction bias, rather than being a nuisance, can be used to improve the predictions. We use this to develop a novel method to predict the response to selection, which is built on three key innovations. First, the method predicts change as the breeder’s equation plus a bias term. Second, the method combines information from the breeder’s equation and from the record of past changes in the mean, to estimate the bias and predict change using a Kalman filter. Third, the parameters of the filter are fitted in each generation using a machine-learning algorithm on the record of past changes. We apply the method to data of an artificial selection experiment of the wing of the fruit fly, as well as to an in silico evolution experiment for teeth. We find that the method outperforms the breeder’s equation, and notably provides good predictions even when traits under selection are omitted from the analysis and when additive genetic variance is estimated inaccurately. The proposed method is easy to apply since it only requires recording the mean of the traits over past generations.
DOI
https://doi.org/10.32942/osf.io/tkq9w
Subjects
Animal Sciences, Computational Biology, Ecology and Evolutionary Biology, Evolution, Genetics, Genetics and Genomics, Life Sciences, Other Ecology and Evolutionary Biology, Population Biology
Keywords
Dates
Published: 2021-09-27 13:51
License
CC-By Attribution-NonCommercial-NoDerivatives 4.0 International
There are no comments or no comments have been made public for this article.