This is a Preprint and has not been peer reviewed. This is version 2 of this Preprint.
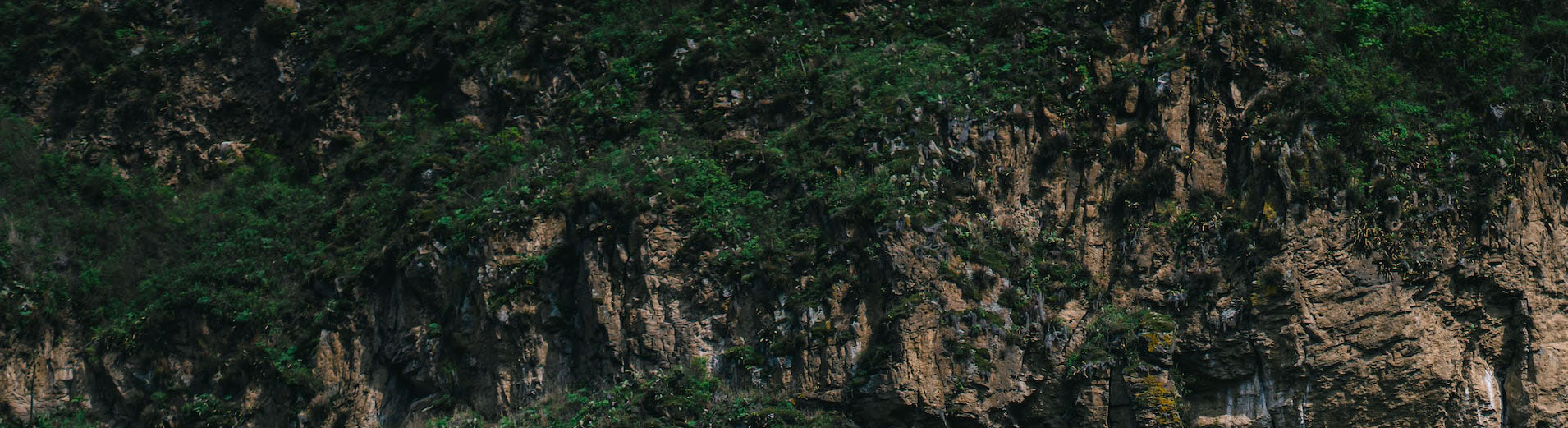
Downloads
Supplementary Files
Authors
Abstract
Ecology and evolutionary biology, like other scientific fields, are experiencing an exponential
growth of academic manuscripts. As domain knowledge accumulates, scientists will need
new computational approaches for identifying relevant literature to read and include in
formal literature reviews and meta-analyses. Importantly, these approaches can also
facilitate automated, large-scale data synthesis tasks and build structured databases from
the information in the texts of primary journal articles, books, grey literature, and
websites. The increasing availability of digital text, computational resources, and
machine-learning based language models have led to a revolution in text analysis and
Natural Language Processing (NLP) in recent years. NLP has been widely adopted across
the biomedical sciences, but is rarely used in ecology and evolutionary biology. Applying
computational tools from text mining and NLP will increase the efficiency of data synthesis,
improve the reproducibility of literature reviews, formalize analyses of research biases and
knowledge gaps, and promote data-driven discovery of patterns across ecology and
evolutionary biology. Here we present recent use cases from ecology and evolution, and
discuss future applications, limitations, and ethical issues.
DOI
https://doi.org/10.32942/osf.io/c4kvq
Subjects
Biodiversity, Bioinformatics, Ecology and Evolutionary Biology, Life Sciences
Keywords
biodiversity science, computational linguistics, database construction, document classification, Information Extraction, Information Retrieval, Named Entity Recognition, natural language processing, NLP, relation extraction, topic model
Dates
Published: 2022-02-16 21:03
Last Updated: 2022-04-05 14:59
There are no comments or no comments have been made public for this article.