This is a Preprint and has not been peer reviewed. This is version 2 of this Preprint.
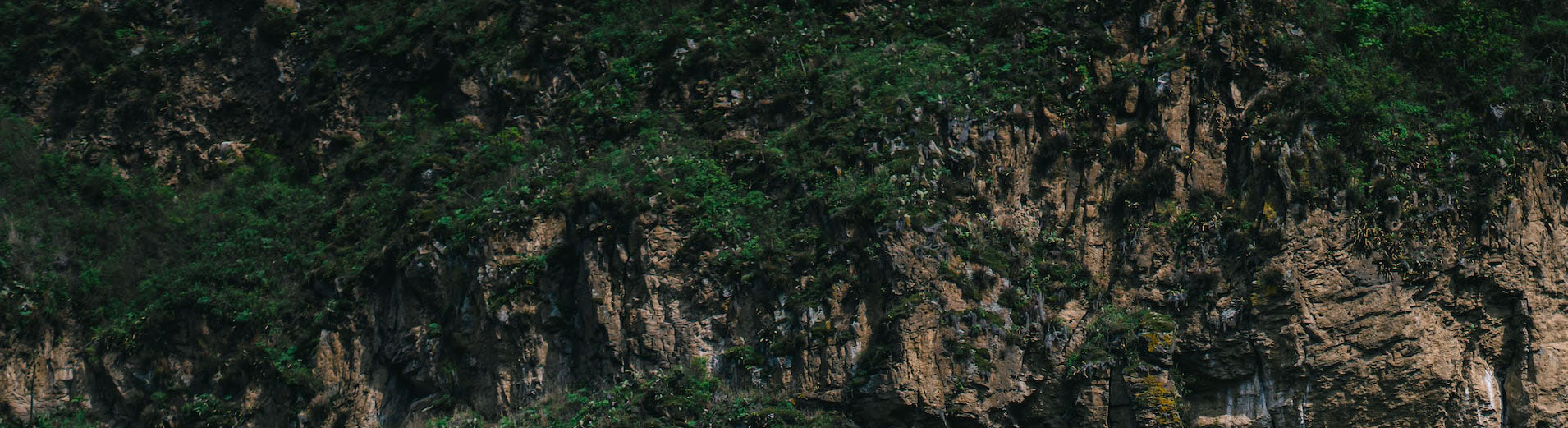
Machine learning pipeline extracts biologically significant data automatically from avian monitoring videos
Downloads
Supplementary Files
Authors
Abstract
Measuring parental care behaviour in the wild is central to the study of animal life-history trade-offs, but is often labour and time-intensive. More efficient machine learning-based video processing tools have recently emerged that allow parental nest visit rates to be measured using video cameras and computer processing. Here, we used open-source software to detect movement events from videos taken at the nest box of a wild passerine bird population. We show that visit numbers from our automatic data collection pipeline strongly correlate with human observations and predicts an increase in brood fitness. Using a machine learning-assisted annotation approach on a subset of 18 videos, we show that the accuracy largely increased and cut annotation time by an average of 5.5x compared to that of a cohort of undergraduate students. Since our automatic pipeline collected biological-meaningful data that would have taken approximately 800 days by human observers, we encourage more researchers to apply existing open-source tools to assist data collection in animal behaviour studies.
DOI
https://doi.org/10.32942/osf.io/zdeqm
Subjects
Behavior and Ethology, Biology, Ecology and Evolutionary Biology, Life Sciences
Keywords
computer vision, Deep Meerkat, House Sparrow, machine learning, parental care
Dates
Published: 2022-03-08 03:14
Last Updated: 2022-04-04 01:10
Older Versions
License
CC-By Attribution-ShareAlike 4.0 International
Additional Metadata
Data and Code Availability Statement:
Available upon request
There are no comments or no comments have been made public for this article.