This is a Preprint and has not been peer reviewed. The published version of this Preprint is available: https://doi.org/10.1111/2041-210X.13901. This is version 1 of this Preprint.
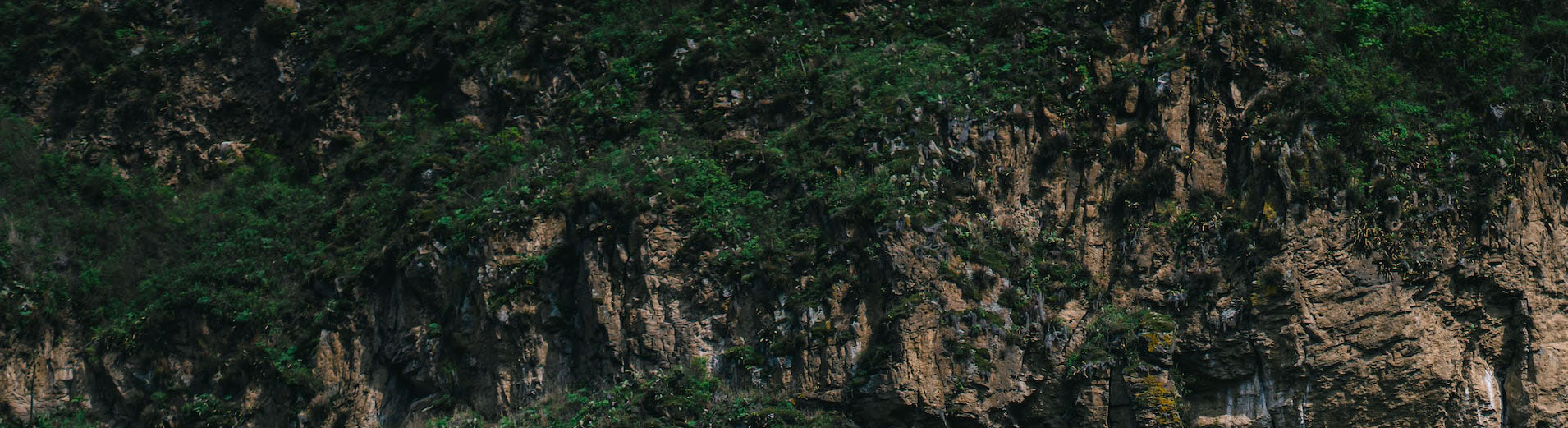
Deep learning as a tool for ecology and evolution
Downloads
Supplementary Files
Authors
Abstract
Deep learning is driving recent advances behind many everyday technologies, including those relying on speech and image recognition, natural language processing, and autonomous driving. It is also gaining popularity in biology, where it has been used for automated species identification, environmental monitoring, behavioral studies, DNA sequencing, and population genetics and phylogenetics, among other applications. Deep learning relies on artificial neural networks for predictive modeling and excels at recognizing complex patterns. Operating within the machine learning paradigm, deep learning can be viewed as an alternative to likelihood-based inference methods. It has desirable properties, including good performance and scaling with increasing complexity, while posing unique challenges such as sensitivity to bias in input data. In this review we provide a gentle introduction to deep learning, review its applications in ecology and evolution, and discuss its limitations and efforts to overcome them. We also provide a practical primer for biologists interested in including deep learning in their toolkit and identify its possible future applications.
DOI
https://doi.org/10.32942/osf.io/nt3as
Subjects
Ecology and Evolutionary Biology, Life Sciences
Keywords
Behavior, Deep learning, ecology, evolution, genomics, machine learning, modeling, phylogenetics, Population genetics, review, species delimitation, statistics
Dates
Published: 2021-06-26 02:13
There are no comments or no comments have been made public for this article.