This is a Preprint and has not been peer reviewed. This is version 2 of this Preprint.
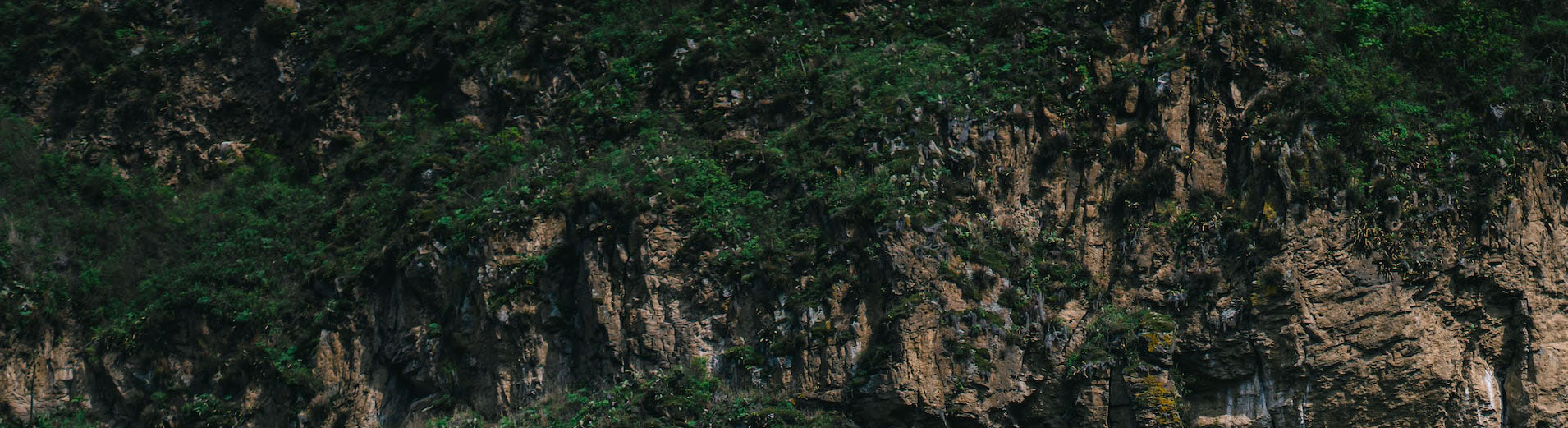
Minimum Sampling, Maximum Insight: Tracking Environmental Trends in a Tidal Estuary
Downloads
Authors
Abstract
Long-term environmental monitoring is essential for detecting ecological trends and managing dynamic systems. In estuarine environments, where monitoring is often constrained by cost and logistics, efficient resource allocation is key to sustaining effective programs. We developed a framework to optimize spatial and temporal sampling in the Great Bay Estuary (New Hampshire/Maine, USA), identifying the minimum number of years and sites needed to detect long-term trends. Using 23 years of data on five water quality parameters from 10 sites, we applied a resampling-based trend detection algorithm to estimate minimum sampling effort. Our results show that trend detectability varies by parameter and location, with each requiring different sampling durations. These thresholds also depend on the user-defined level of statistical power (e.g., 80% vs. 100%). For example, nitrogen trends were detectable with as few as five years of data, while dissolved oxygen required up to seven. Additionally, 8–9 sites were sufficient to achieve 80% statistical power, suggesting spatial redundancy at some locations. Variance partitioning revealed that autocorrelation, slope error, and data variability each influenced sampling effort for reliable trend detection. Parameters such as dissolved oxygen and water temperature—both highly autocorrelated—required longer time series, while those with lower slope precision, like nitrogen, were sensitive to measurement error. These findings underscore the value of adaptive monitoring designs that align sampling strategies with the statistical and ecological characteristics of individual parameters. Our approach provides a flexible, data-driven framework for refining estuarine monitoring programs, enabling managers to maintain ecological insight while optimizing resource use.
DOI
https://doi.org/10.32942/X2Z056
Subjects
Environmental Monitoring, Environmental Sciences, Water Resource Management
Keywords
Environmental monitoring, estuarine systems, trend detection, sampling optimization, statistical power
Dates
Published: 2025-05-23 15:08
Last Updated: 2025-05-23 15:08
Older Versions
License
CC BY Attribution 4.0 International
Additional Metadata
Conflict of interest statement:
This work was supported by the Piscataqua Region Estuaries Partnership (PREP), whose data were used in the study. Co-authors Barbara Spiecker and Easton White received research funding from PREP. Co-authors Kalle Matso and Chris Whitney are employed by PREP as Director and Data Scientist, respectively. PREP receives funding from the U.S. Environmental Protection Agency and also from municipalities in the Piscataqua Region Watershed.
Data and Code Availability Statement:
The data that support the findings of this study are openly available in Piscataqua Watershed Data Explorer at http://data.prepestuaries.org:8510/. All code used in this study is available at https://github.com/QuantMarineEcoLab/prep-sampling-optimization.
Language:
English
There are no comments or no comments have been made public for this article.