This is a Preprint and has not been peer reviewed. This is version 2 of this Preprint.
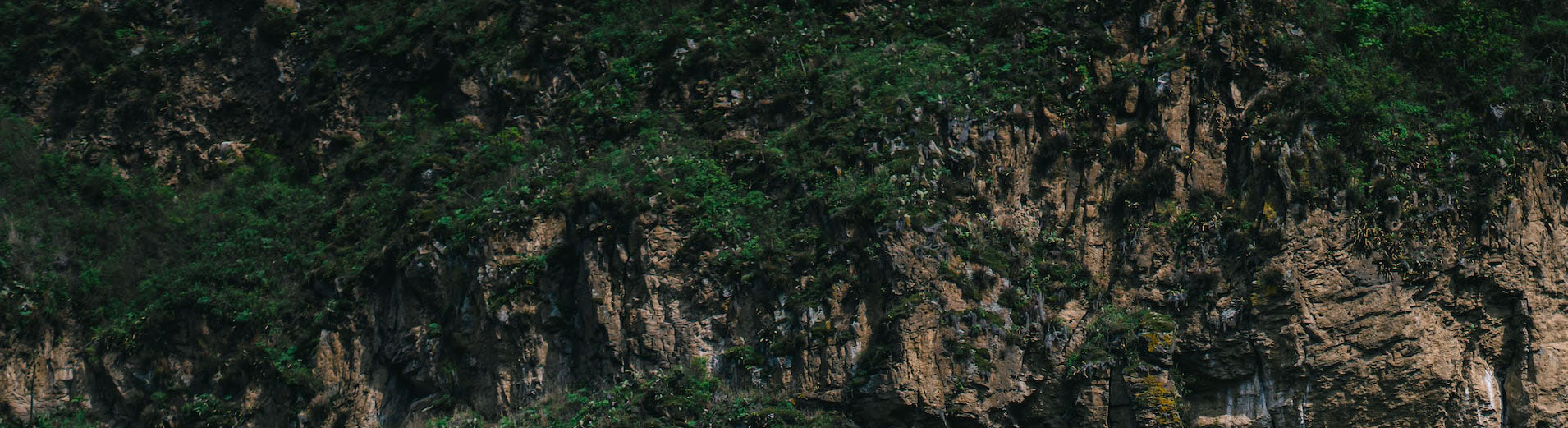
Filling Monitoring Gaps for Data-deficient Species Using Annual Occupancy Predictions from Co-occurrence Models
Downloads
Authors
Abstract
Fragmented surveys and limited monitoring exclude most invertebrate species from conservation policy. We present a framework that generates annual occupancy predictions using species distribution models (SDMs) to reconstruct missing trends—not to extrapolate trends, but to fill them in (the fill-in approach). Instead of filtering poor-data regions and years or relying on static environmental variables, we use co-occurrence patterns (COP) to capture year-to-year shifts in species assemblages, to enable temporal prediction across all recorded habitats using sparse, presence-only datasets from multiple sources. Applied to four rare native ladybugs across North America (2007–2021), COP models exceeded reliability benchmarks (Accuracy > 0.70, AUC > 0.70, Kappa > 0.40, Brier < 0.25) across standard test splits, structurally distinct sources, and temporally divided periods. This indicates that annual predictions were robust to temporal bias arising from varying data volume and source composition, as supported by negligible effects in multiple regression. Predicted 10-year declines (9–31%) closely aligned with independent long-term regional monitoring data, operationalizing IUCN Red List classifications (from “Least Concern” to “Vulnerable”) in the absence of standardized monitoring. By translating fragmented observations—primarily from citizen science—into standardized annual trend estimates, the fill-in approach extends extinction risk assessment to data-deficient taxa long excluded from conservation frameworks.
DOI
https://doi.org/10.32942/X2KS8V
Subjects
Biodiversity, Ecology and Evolutionary Biology, Entomology, Life Sciences
Keywords
Data Deficient, extinction risk, citizen science, Multi-source, Structural Bias, Temporal Bias, Adventive Species, Native Ladybug Decline
Dates
Published: 2025-05-21 06:18
Last Updated: 2025-06-05 02:26
Older Versions
License
CC BY Attribution 4.0 International
Additional Metadata
Conflict of interest statement:
The authors declare no conflict of interest.
Data and Code Availability Statement:
A code package designed for ease of public use will be made available with official publication. Current version: https://figshare.com/s/36131cf2516dc300e80a?file=54689660
Language:
English
There are no comments or no comments have been made public for this article.