This is a Preprint and has not been peer reviewed. This is version 1 of this Preprint.
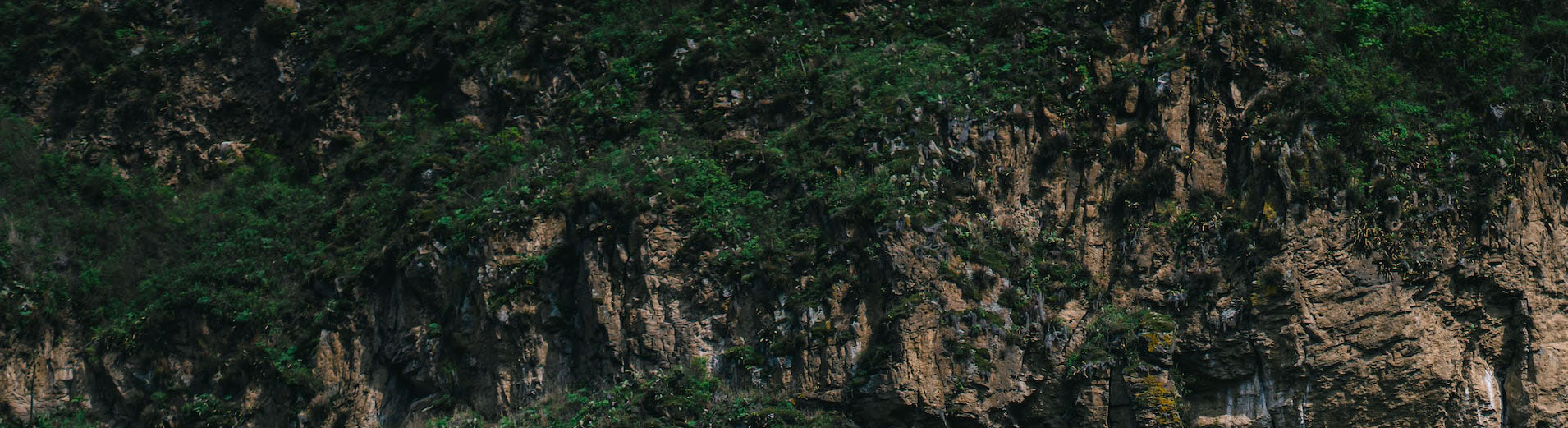
Using large language models to address the bottleneck of georeferencing natural history collections
Downloads
Authors
Abstract
Natural history collections are fundamental for biodiversity research. The broad use of them relies on the digitization effort, especially georeferencing that translates textual locality descriptions into geographic coordinates. However, traditional georeferencing approaches are labor-intensive and costly, thus georeferencing is a major bottleneck in the digitization process that prevents the usage of millions of specimens across the world. This study investigated the potential of using large language models (LLMs) to facilitate georeferencing. We utilized LLMs from OpenAI and DeepSeek to georeference 5,000 vascular plant specimen records with known coordinates, and compared the results against those of GEOLocate (a widely used georeferencing tool) and manual georeferencing. We found that the best-performing LLMs (e.g., gpt-4o) outperformed specialized tools like GEOLocate in spatial applicability, and demonstrated near-human-level accuracy with a median georeferencing error of <10 km. Georeferencing based on LLMs were also considerably fast (<1 s per record) and affordable ($0.10 per 100 records); thus, they present a cost-effective approach for georeferencing. LLMs may not fully replace human curation in the short term, but can be incorporated into current workflows to greatly increase the efficiency of georeferencing. Future advances in LLMs may revolutionize the digitization of natural history collections.
DOI
https://doi.org/10.32942/X2134G
Subjects
Biodiversity, Ecology and Evolutionary Biology
Keywords
Artificial Intelligence, Large Language Model, biodiversity, herbarium, museum, Specimen
Dates
Published: 2025-05-02 15:58
Last Updated: 2025-05-02 15:58
License
CC BY Attribution 4.0 International
Additional Metadata
Language:
English
There are no comments or no comments have been made public for this article.