This is a Preprint and has not been peer reviewed. This is version 1 of this Preprint.
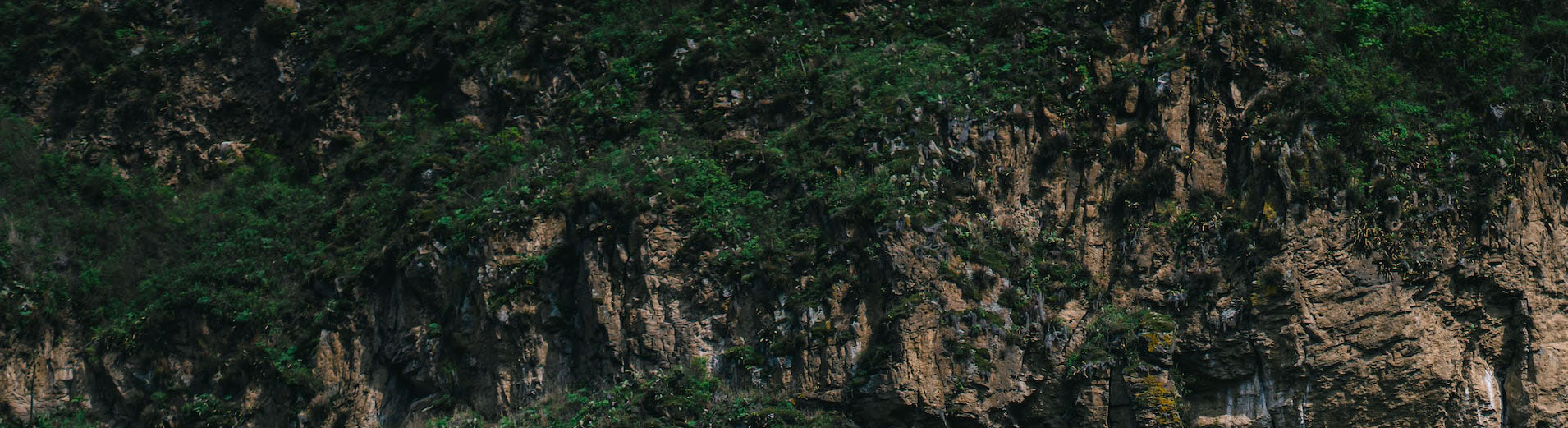
Improving landscape fire frequency estimates by integrating public land fire data and satellite imagery
Downloads
Authors
Abstract
Background
Contemporary fire regimes are changing rapidly, and effective fire management requires knowledge of fire history, often derived from satellite imagery. Satellites, however, are not well suited to detecting low intensity fires, meaning fire history data are often inaccurate.
Aims
We aimed to improve satellite fire frequency estimates by incorporating data from fire history on public land and environmental co-variation.
Methods
Using a generalisable workflow, we applied boosted regression trees, generalised linear, and generalised additive models to predict fire frequency in an eastern Australia case study.
Key results
Relative to unprocessed satellite data, generalised linear and generalised additive models improved correlations with public land fire data by 0.39 and 0.25, respectively. Generalised linear models estimated low fire frequencies well (≤2 fires), whereas generalised additive models predicted higher fire frequencies (≥3 fires) more accurately.
Conclusions
For mapping land as burnt or unburnt, generalised linear models are most appropriate. For understanding the total number of fires over time, and for most vegetation types, predictions from generalised additive model are most appropriate.
Implications
Our approach can improve the accuracy of fire frequency estimates from satellite data, to assist fire management and conservation. However, model selection will depend on the application and vegetation type.
DOI
https://doi.org/10.32942/X24331
Subjects
Ecology and Evolutionary Biology
Keywords
fire management, fire scar mapping, Landsat, predicitive modelling, satellite fire data, Sentinel, species distribution modelling, remote sensing
Dates
Published: 2025-04-23 14:14
Last Updated: 2025-04-23 14:14
License
CC-BY Attribution-NonCommercial 4.0 International
Additional Metadata
Language:
English
Data and Code Availability Statement:
Open data/code has been made available for peer-review only as an archived Zenodo repository (Charles and Smith 2025) https://doi.org/10.5281/zenodo.15133643.
There are no comments or no comments have been made public for this article.