This is a Preprint and has not been peer reviewed. This is version 1 of this Preprint.
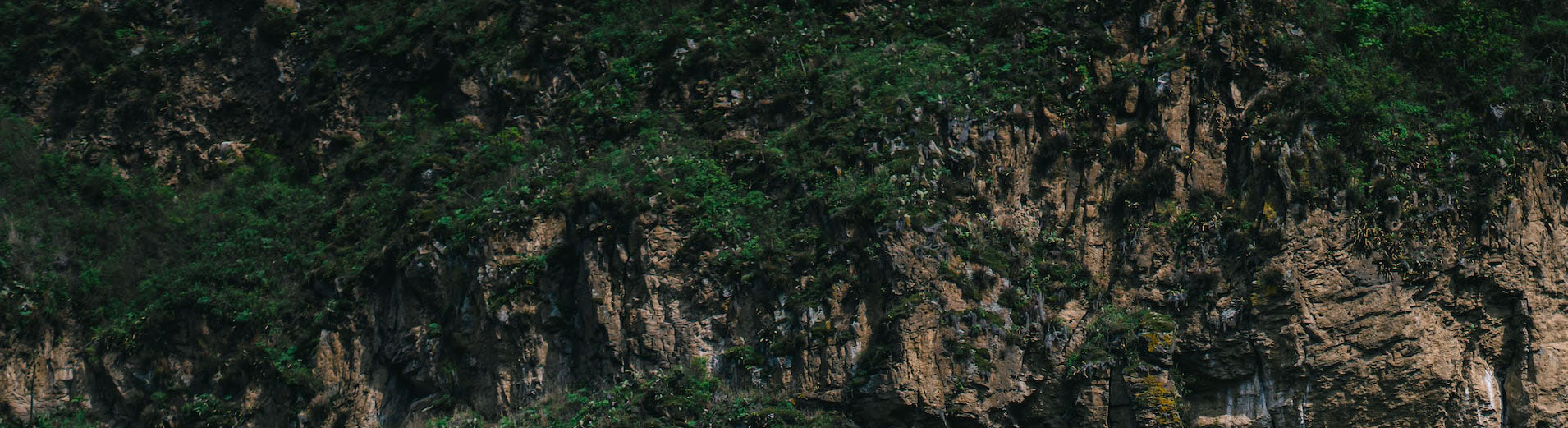
Leveraging large language models for ecological interpretation using an eBird chatbot case study
Downloads
Authors
Abstract
1. The anthropocene presents significant challenges for global biodiversity, public health, and long-term ecosystem stability. The wealth of publicly available near-real-time ecology and climate data can be used to monitor these challenges and allow practitioners to develop mitigation strategies.
2. There is untapped potential to apply Large Language Models (LLMs) to quantitative ecological and environmental datasets, enabling researchers and practitioners to use natural language queries to transform ecological observations into actionable insights for both conservation action and external communication of results to diverse audiences. Advances in artificial intelligence (AI), and particularly in LLMS, offer emerging opportunities to address these challenges. LLMs are increasingly proficient at identifying patterns and semantic relationships within textual data, and are highly customisable. Accessible AI tools can also facilitate communication across research and policy sectors.
3. Here, we present a roadmap for designing and implementing multi-modal LLMs to answer ecological research questions. In order to build ‘virtual statistician’ systems capable of fast-tracking data interpretation, we advocate for strategic planning, data stewardship practices, careful prompt-engineering, and model evaluation as key steps in the LLM development process.
4. We showcase a case study that applies the open-source LangChain framework to analyse citizen science data using the eBird database to produce a chatbot allowing the user to ask quantitative questions about near-real-time bird observations. Using our LLM roadmap, we highlight the importance of iterative and strategic prompt engineering and agent selection, in addition to iteratively evaluating model output.
5. As LLM software continues to evolve, their integration into ecological and environmental research can empower ecologists with purpose-built tools that bridge the gap between data collection and actionable solutions.
DOI
https://doi.org/10.32942/X2CH1K
Subjects
Biodiversity, Bioinformatics, Ecology and Evolutionary Biology
Keywords
large language models, citizen science, Artificial intelligence, natural language processing, multi-agent models, llms, citizen science, Artificial Intelligence, natural language processing, multi-modal models, multi-agent models
Dates
Published: 2025-04-23 13:07
Last Updated: 2025-04-23 13:07
License
CC BY Attribution 4.0 International
Additional Metadata
Conflict of interest statement:
None
Data and Code Availability Statement:
Scripts and data used to conduct the eBird chatbot case study are publicly available for review and download at: https://github.com/BioDivHealth/eBird_testing
Language:
English
There are no comments or no comments have been made public for this article.