This is a Preprint and has not been peer reviewed. This is version 1 of this Preprint.
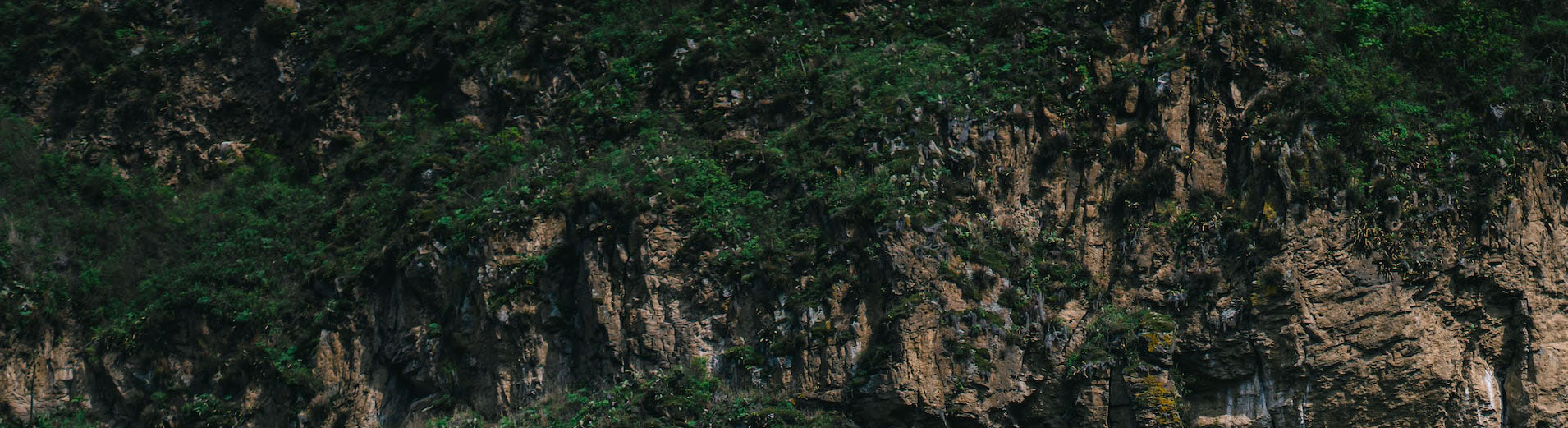
Quantifying the correlation between variance components: an extension to the double-hierarchical generalised linear model
Downloads
Authors
Abstract
1. The variational properties of biological systems are an increasing focus of current research, and statistical methods are required for drawing inferences about the processes that determine them.
2. Double-hierarchical generalised linear models (DHGLM) are ideally suited for studying variational properties since they provide a direct way of modelling the distribution of variances.
3. Although DHGLM have mainly been used to model heterogeneous residual variances over groups, models have been proposed that also allow heterogeneous random effect variances. However, these multi-way DHGLM make the assumption that the residual variance of a group is independent of its random effect variance. Here, using a Bayesian approach, we extend multi-way DHGLMs so that the correlation between residual and random-effect variances can be estimated.
4. Using simulated data, the performance of the model is compared with the non-DHGLM models that have traditionally been used to estimate such correlations. The proposed model is shown to perform well at estimating all model parameters, and in particular performs better than alternative models at estimating the correlation among variance components.
5. Numerical analyses are complemented with theoretical work showing the expected bias when using non-DHGLM models. In some cases, commonly-used non-DHGLM models are even expected to get the sign of the correlation wrong.
DOI
https://doi.org/10.32942/X2ZH1V
Subjects
Life Sciences
Keywords
variance decomposition, double-hierarchical, heritability, Repeatability
Dates
Published: 2025-04-14 08:58
Last Updated: 2025-04-14 08:58
License
Additional Metadata
Conflict of interest statement:
None.
Data and Code Availability Statement:
Not applicable.
Language:
English
There are no comments or no comments have been made public for this article.