This is a Preprint and has not been peer reviewed. This is version 1 of this Preprint.
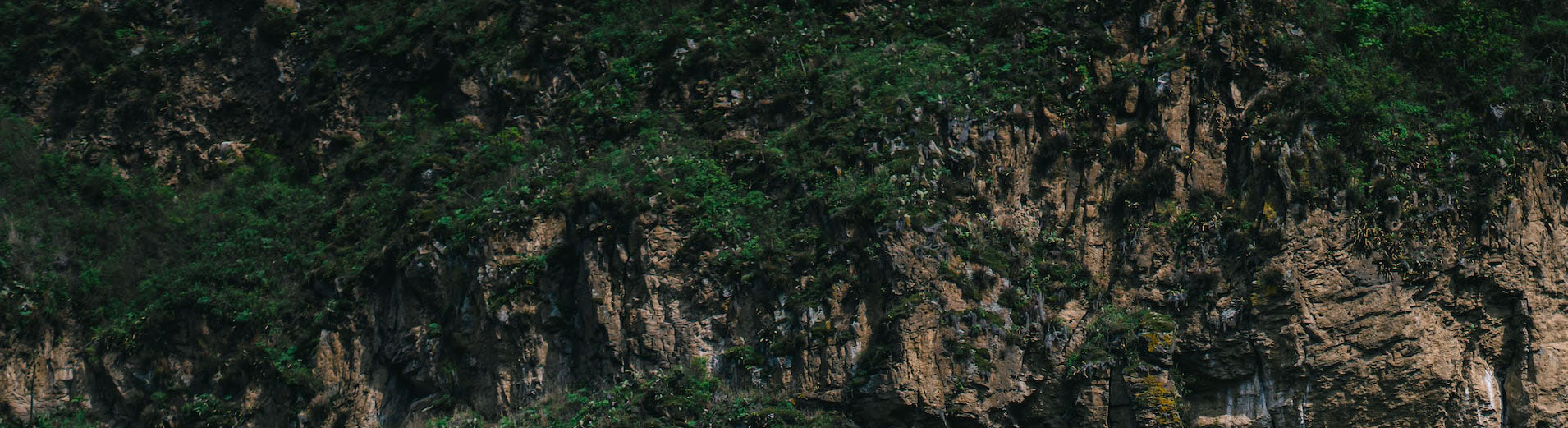
Modelling approaches for meta-analyses with dependent effect sizes in ecology and evolution: A simulation study
Downloads
Supplementary Files
Authors
Abstract
1. In ecology and evolution, meta-analysis is an important tool to synthesise findings across separate studies. However, ecological and evolutionary data often exhibit complex dependence structures, such as shared sources of variation within studies, phylogenetic relationships, and hierarchical sampling designs. Recent statistical advancements offer approaches for handling such complexities in dependence, yet these methods remain underutilised or unfamiliar to ecologists and evolutionary biologists.
2. We conducted extensive simulations to evaluate modelling approaches for handling dependence in effect sizes and sampling errors in ecological and evolutionary meta-analyses. We assessed the performance of multilevel models, incorporating an assumed sampling error variance-covariance matrix (which account for within-study correlation), cluster robust variance estimation (CRVE) methods and their combination across different true within-study correlations. Finally, we showcased the applications of these models in two case studies of published meta-analyses.
3. Multilevel models produced unbiased regression coefficient estimates and when a sampling variance-covariance matrix was used it provided accurate random effect variance components estimates within and among studies. However, the latter had no impact on regression coefficient estimates if the model was misspecified. The inclusion of CRVE methods, either alone or combined with multilevel models, did not enhance performance. In simulations involving phylogenetic multilevel meta-analysis, models using CRVE methods generated narrower confidence intervals and lower coverage rates than the nominal expectations. The case study results showed the importance of considering a sampling error variance-covariance matrix to improve the model fit.
4. Our results provide clear modelling recommendations for ecologists and evolutionary biologists conducting meta-analyses. To improve the precision of variance component estimates we recommend constructing a variance–covariance matrix that accounts for dependencies in sampling errors within studies. Although CRVE methods provide robust inference under certain conditions, we caution against their use with crossed random effects, such as phylogenetic multilevel meta-analyses, as CRVE methods currently do not account for multi-way clustering and may inflate Type I error rates. Finally, we recommend using multilevel meta-analytic models to account for heterogeneity at all relevant hierarchical levels and to follow guidance on inference methods to ensure accurate coverage of the overall mean.
DOI
https://doi.org/10.32942/X2RW6P
Subjects
Applied Statistics, Ecology and Evolutionary Biology, Other Ecology and Evolutionary Biology, Statistical Methodology
Keywords
Non-independence, Sandwich estimators, Sandwich estimators, mixed-effects models, multi-species, meta-regression, Phylogenetic comparative methods, Cross-classified data
Dates
Published: 2025-04-08 16:36
Last Updated: 2025-04-08 16:36
License
CC-By Attribution-NonCommercial-NoDerivatives 4.0 International
Additional Metadata
Language:
English
Conflict of interest statement:
None
There are no comments or no comments have been made public for this article.