This is a Preprint and has not been peer reviewed. This is version 1 of this Preprint.
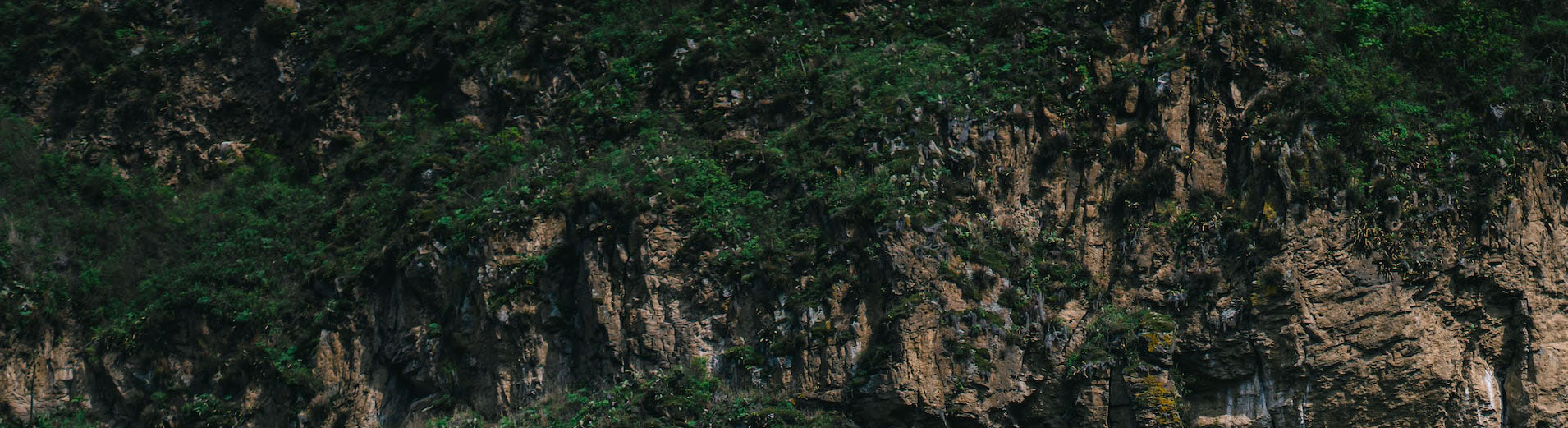
Reflections from the 2025 EcoHack: AI & LLM Hackathon for Applications in Evidence-based Ecological Research & Practice
Downloads
Authors
Abstract
This paper presents outcomes from the inaugural "EcoHack: AI & LLM Hackathon for Applications in Evidence-based Ecological Research & Practice," which convened participants from across Europe and beyond, culminating in 11 team submissions. These submissions highlighted six broad application areas of AI for ecology: (1) AI-enhanced decision support and automation, (2) scientific search and communication, (3) knowledge extraction and reasoning, (4) AI for ecological modeling, forecasting, and simulation, (5) causal inference and ecological reasoning, and (6) AI for biodiversity monitoring and conservation. Each team’s project is summarized in a consolidated table—complete with links to source code—and described in brief papers in the appendix.
Beyond summarizing technical results, this paper offers insights into the hackathon’s hybrid structure, featuring an in-person gathering in Bielefeld, Germany, alongside a global online hub that facilitated both local and virtual engagement. Throughout the event, participants showcased how large language models (LLMs) can serve as both robust tools for diverse machine learning tasks and flexible platforms for rapidly prototyping novel research applications. These efforts underscore the importance of stronger technological bridges among stakeholders in ecology, including practitioners, local farmers, and policymakers. Overall, the EcoHack outcomes highlight the transformative potential of AI in driving scientific discovery and fostering interdisciplinary collaboration in ecology.
DOI
https://doi.org/10.32942/X2XP7J
Subjects
Computer Sciences, Ecology and Evolutionary Biology, Forest Sciences
Keywords
AI for Ecology, Large Language Models (LLMs), ecological modeling, Hackathon, Scientific Knowledge Extraction, Conservation and biodiversity, Decision-making tools for policymakers, Multi-agent simulations, Ontology mapping
Dates
Published: 2025-04-05 17:57
Last Updated: 2025-04-05 17:57
License
CC-By Attribution-ShareAlike 4.0 International
Additional Metadata
Language:
English
Data and Code Availability Statement:
https://github.com/EcoWeaver/EcoHack/
There are no comments or no comments have been made public for this article.