This is a Preprint and has not been peer reviewed. This is version 2 of this Preprint.
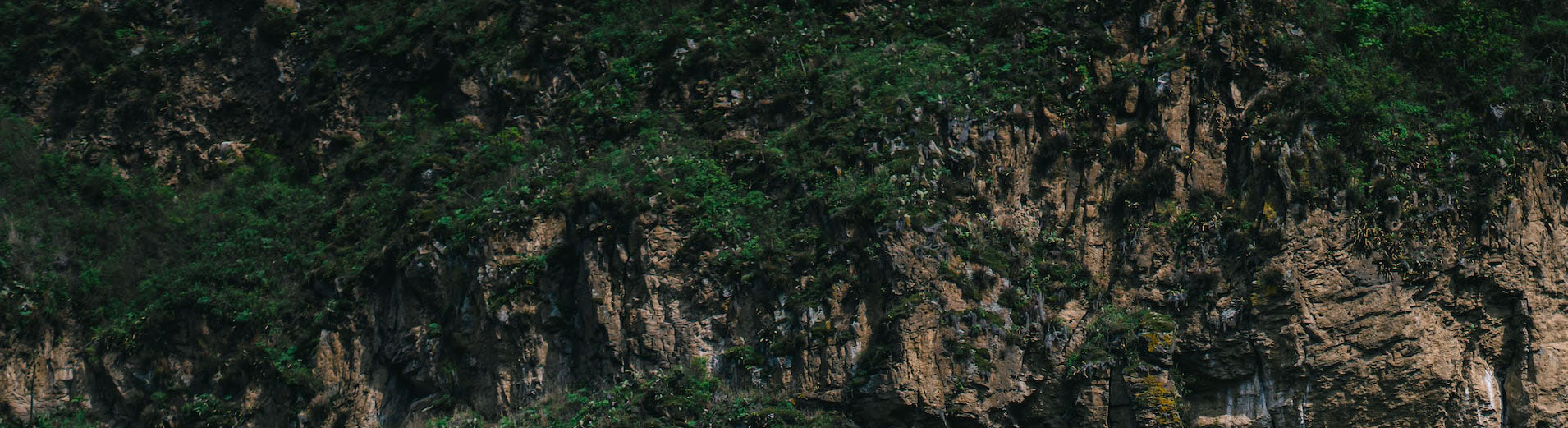
HusMorph: A simple machine learning app for automated morphometric landmarking
Downloads
Authors
Abstract
Manually obtaining the length and other morphometric features of an animal can be time- consuming, and consistent measurements are challenging with large datasets. By leveraging high-throughput computing power and machine learning-based computer vision, such phenotypic data can be rapidly collected with high accuracy. Here we present HusMorph, a novel application with a simple and intuitive graphical user interface (GUI), based on the same machine learning method used in other pipelines such as ML-morph. It consists of an all-in-one package with the goal of making machine learning easy to use for non-experts. The user starts by setting any number of landmarks on a set of photos captured with a standardized setup. From this set, a machine learning model is generated by automatically and randomly searching for the best performing parameters. Next, the user can apply the model to predict landmarks on new standardized photos, and visually confirm and export the results of the predictions. For measuring length between landmarks, an additional feature allows for detecting a scale bar for each photo to convert the length from pixels to a metric unit. Our application has been validated and applied to extract standard length from 1,935 photos of zebrafish and performs with about 99.5% accuracy compared to manual measurements along with 100% scale bar detection.
DOI
https://doi.org/10.32942/X2F92H
Subjects
Artificial Intelligence and Robotics, Biology
Keywords
Artificial Intelligence, automation, images, morphometrics, Phenotyping, User-friendly
Dates
Published: 2025-02-21 14:39
Last Updated: 2025-02-26 14:50
Older Versions
License
Additional Metadata
Language:
English
Data and Code Availability Statement:
The code repository for the project are openly available at GitHub: https://github.com/HenHus/Husmorph
There are no comments or no comments have been made public for this article.