This is a Preprint and has not been peer reviewed. This is version 2 of this Preprint.
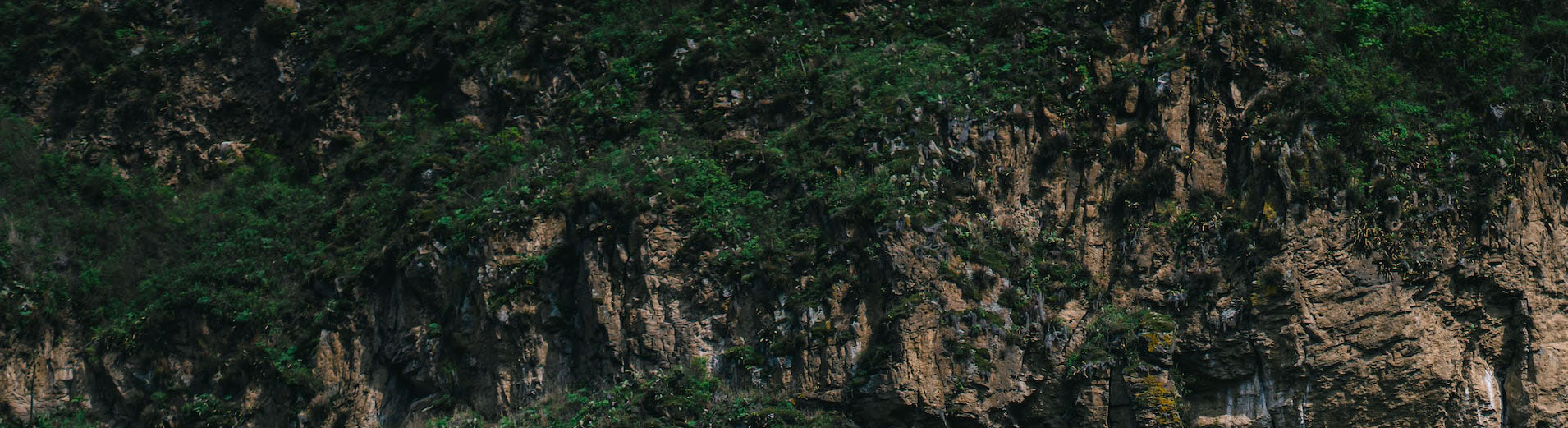
Downloads
Authors
Abstract
Ecosystem maps support a vast array of applications in conservation, land management and policy. The capacity of an ecosystem map to support these applications is determined by its ability to accurately represent ecosystem distributions, which is heavily influenced by the model used to produce them. Here, we evaluated the influence of key modelling decisions made whilst developing a new and comprehensive ecosystem map using a recently developed ecosystem typology for the remote Tiwi Islands, Australia. We collated a reference set of training points from diverse datasets and employed a pixel-based, random forest model to classify and predict ecosystem distributions. We tested decisions at three stages of the model formulation. First, we tested the number of classes by aggregating ecosystem types (finest scale, n = 11) into functional groups (n = 10) and biomes (coarsest, n = 8) according to the Global Ecosystem Typology. Second, we compared data acquired from the Sentinel-2 satellite using the MSI sensor and Landsat-9 with the OLI-2 sensor. Finally, we tested covariates from satellite image bands only or satellite imagery combined with additional covariates describing other ecological characteristics. We evaluated these decisions using a range of model performance metrics, including overall, by-class and spatially explicit estimates. Our study found that using covariates additional to those from satellite images improved all evaluation metrics for all model decisions. Acquisitions from Landsat-9 tended to improve model performance over Sentinel-2, although the effect was variable. Developing maps at the biome scale (coarsest resolution) slightly improved overall performance but hinders applications that need to differentiate between ecosystem types. Including additional relevant covariates or considering alternative satellites are better options for improving map performance than simplifying the classes. Producing spatially explicit evaluation of ecosystem maps is a rapid and achievable method to communicate limitations and support users to make informed decisions.
DOI
https://doi.org/10.32942/X2VK7W
Subjects
Ecology and Evolutionary Biology, Other Ecology and Evolutionary Biology
Keywords
remote sensing, Earth Observation, Vegetation mapping, land cover, island ecology, tropical savanna, machine learning, biogeography
Dates
Published: 2025-02-05 10:29
Last Updated: 2025-02-07 08:57
Older Versions
License
CC BY Attribution 4.0 International
Additional Metadata
Language:
English
Conflict of interest statement:
None
Data and Code Availability Statement:
Data and code will be made publicly available upon acceptance of the manuscript.
There are no comments or no comments have been made public for this article.