This is a Preprint and has not been peer reviewed. This is version 1 of this Preprint.
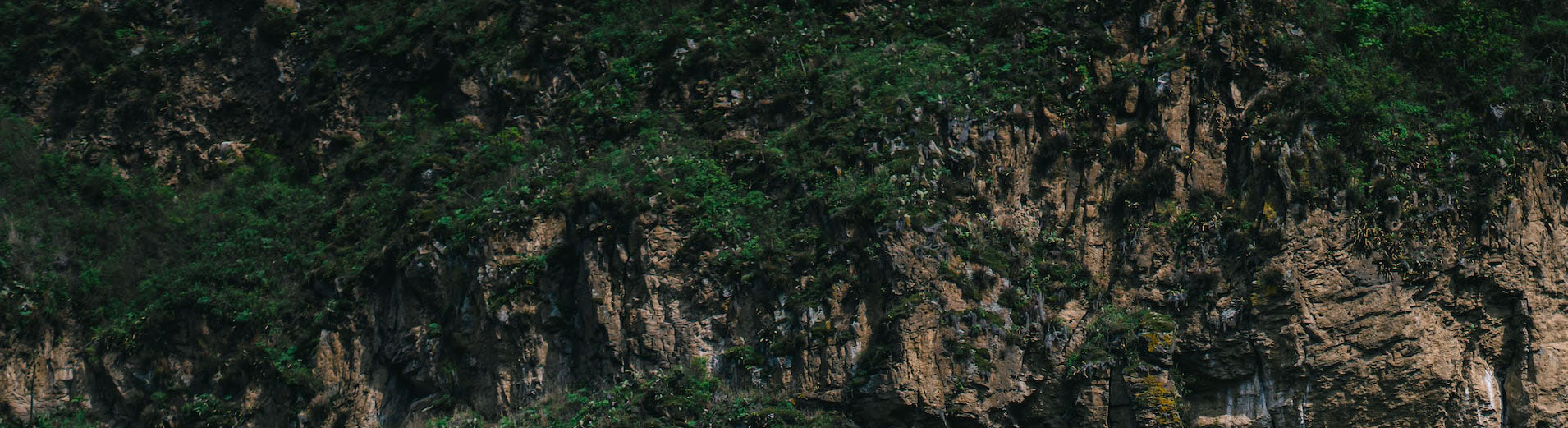
Downloads
Authors
Abstract
1. Motivation: Artificial Intelligence (AI) can rapidly process large ecological datasets, uncover patterns, and inform conservation decisions. However, its adoption by ecologists is often hindered by steep learning curves, overwhelming model options with varying transparency, and uneven access to data, code, and technical skills. We led a workshop, EcoViz+AI: Visualization and AI for Ecology, that brought together 35 experts to synthesize this review and related resources that collectively aim to guide ecologists as they navigate, implement, interpret, and contribute to the fast-evolving AI landscape.
2. Methods: Workshop facilitators led discussions and collaborative coding sessions around five use cases of AI in ecology for processing image, ecophysiological, and acoustic data. Using workshop discussions and experiences as a foundation, this review article synthesizes the opportunities and risks for AI in ecology as well as practical challenges and solutions for adopting AI.
3. Outcomes: Ethical and scientifically sound use of AI requires human review, interpretable methods, and greater technical literacy to minimize risks. However, practical challenges more often prevent adoption than ethical concerns. Four solutions include: (1) educational resources to help researchers assess the opportunity cost associated with AI compared to traditional methods, (2) communities of practice to combat the overwhelming landscape of AI with knowledge, technical skills, collaboration, and inclusivity, (3) effective visualizations to address the transparency deficit of AI for understanding and communicating results including model outputs, performance, and functionality, and (4) computational resources to ease the implementation burden of AI through shared data, modifiable code, and accessible computing. Our workshop compiled resources, including science communication videos for five AI use cases and repositories for ecology-related AI models and communities of practice.
4. Synthesis: Cultural shifts towards formal incentivization of open-access educational materials, inclusive mentorship, science communication, and open science will empower ecologists to leverage AI responsibly. Aligning AI initiatives with broader movements towards interdisciplinary open science and computational literacy will promote inclusivity and the ecological relevance of novel tools, advancing basic research and impactful translational ecology.
DOI
https://doi.org/10.32942/X2FK8J
Subjects
Computer Sciences, Ecology and Evolutionary Biology
Keywords
AI, Artificial Intelligence, ecology, education, cyberinfrastructure, Visualization, Science Communication, communities of practice
Dates
Published: 2025-01-29 19:41
License
CC BY Attribution 4.0 International
Additional Metadata
Language:
English
Data and Code Availability Statement:
Not applicable.
There are no comments or no comments have been made public for this article.