This is a Preprint and has not been peer reviewed. This is version 1 of this Preprint.
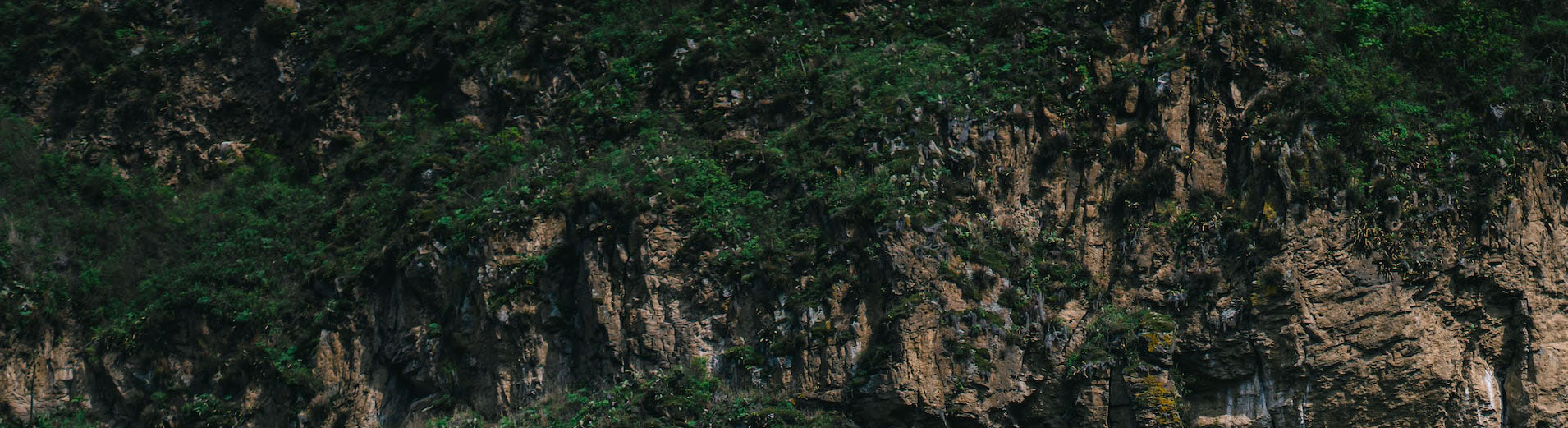
AI and Big Data for invasion biology: finding, modelling and forecasting the population dynamics of invaders
Downloads
Authors
Abstract
Artificial intelligence (AI) is rapidly transforming the study and management of invasive species through analytical and predictive tools that optimize detection, monitoring, and automated eradication. In this work, we reviewed the fundamental principles of machine learning and deep learning, illustrated with recent case studies on invasive species. We also present the first systematic review of AI applications in the field of biological invasions and demography, encompassing 278 articles published since 1999, with 50% of them appearing in the last five years, highlighting the rapid progress in this area and its applications. We observe that most studies focused on plants and detection tasks, utilizing satellite images, drones, and digital cameras as primary data sources, enabling unprecedented precision and efficiency in monitoring invasions. Deep learning algorithms stand out for their ability to process complex visual data, while ensemble modelling approaches produce more robust predictions. The growing availability of global databases, images, and collaborative platforms has significantly reduced fieldwork costs, improved access to larger and remote areas, and enabled the use of algorithms without requiring advanced programming expertise. This work serves as a practical and accessible guide for researchers new to AI, highlighting the most recent advances and its transformative potential to address the challenges of biological invasions.
DOI
https://doi.org/10.32942/X2Q62Z
Subjects
Biodiversity, Bioinformatics, Life Sciences, Population Biology
Keywords
Artificial Intelligence, Demography, exotic species, machine learning, remote sensing, species distribution models
Dates
Published: 2025-01-07 03:43
License
CC BY Attribution 4.0 International
Additional Metadata
Conflict of interest statement:
None
Data and Code Availability Statement:
Will be uploaded upon acceptance in a journal
Language:
espanol
There are no comments or no comments have been made public for this article.