This is a Preprint and has not been peer reviewed. This is version 1 of this Preprint.
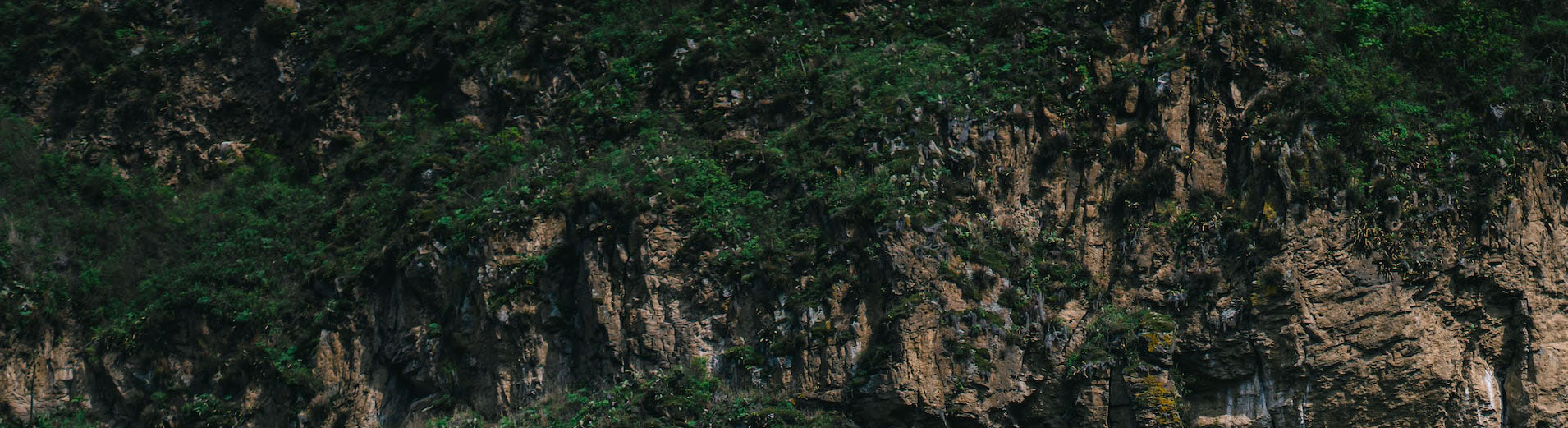
From Vegetation to Vulnerability: Integrating Remote Sensing and AI to Combat Cheatgrass-Induced Wildfire Hazards in California
Downloads
Authors
Abstract
Wildfire risk is on the rise around the world. In places like California, this risk is further instigated by the invasive species
cheatgrass (Bromus tectorum). Cheatgrass is highly flammable and benefits from wildfires, allowing it to replace native plant communities. Through increasing both the intensity and the frequency of wildfires, it endangers not only its natural environment but also human habitats. Here, we present a novel approach to map the distribution and expansion of cheatgrass and predict potential wildfire risk zones. Utilizing the open-source CalFlora dataset, alongside data from the Sentinel–2 satellites, we created a comprehensive spatial analysis framework. We integrated temporal dynamics via Vegetation Index statistical bands that encapsulate annual vegetation information. We
employed semi-supervised learning techniques to refine and filter
our data labels, thereby ensuring robust model training. We utilized machine learning algorithms Random Forest and XGBoost for model training. Our models exhibited a test accuracy of 91.1% in multiclass classification and achieved a precision rate of 91% specifically for the Cheatgrass class. Our multiclass classification model demonstrates exceptional discriminative ability and agreement with the actual classifications, with an ROC-AUC Score of 0.99 indicating near-perfect performance in distinguishing between the different classes, and a Cohen’s Kappa of 0.89 signifying a strong agreement, accounting for chance. We demonstrate the efficacy of our methodology in identifying
regions at high risk of wildfires due to Cheatgrass proliferation,
highlighting the potential for broader application across California’s diverse landscapes. Our analysis effectively predicts the distribution of Cheatgrass and other vegetation with data available only until June, providing insight before the peak forest fire season, which spans from mid-July to September. This capability delivers actionable intelligence for assessing fuel load and connectivity, thus laying the groundwork for targeted wildfire prevention strategies and enhanced ecological management practices in fire-prone areas.
DOI
https://doi.org/10.32942/X2XW67
Subjects
Computational Engineering, Environmental Indicators and Impact Assessment
Keywords
Land use and Land cover Mapping, Cheatgrass, In- vasive Plants, machine learning, Semi-supervised Learning, Wildfire Prevention, Time-series Analysis.
Dates
Published: 2024-12-18 11:50
Last Updated: 2024-12-18 19:50
License
Additional Metadata
Language:
English
Data and Code Availability Statement:
Open data/code are not available.
There are no comments or no comments have been made public for this article.