This is a Preprint and has not been peer reviewed. This is version 1 of this Preprint.
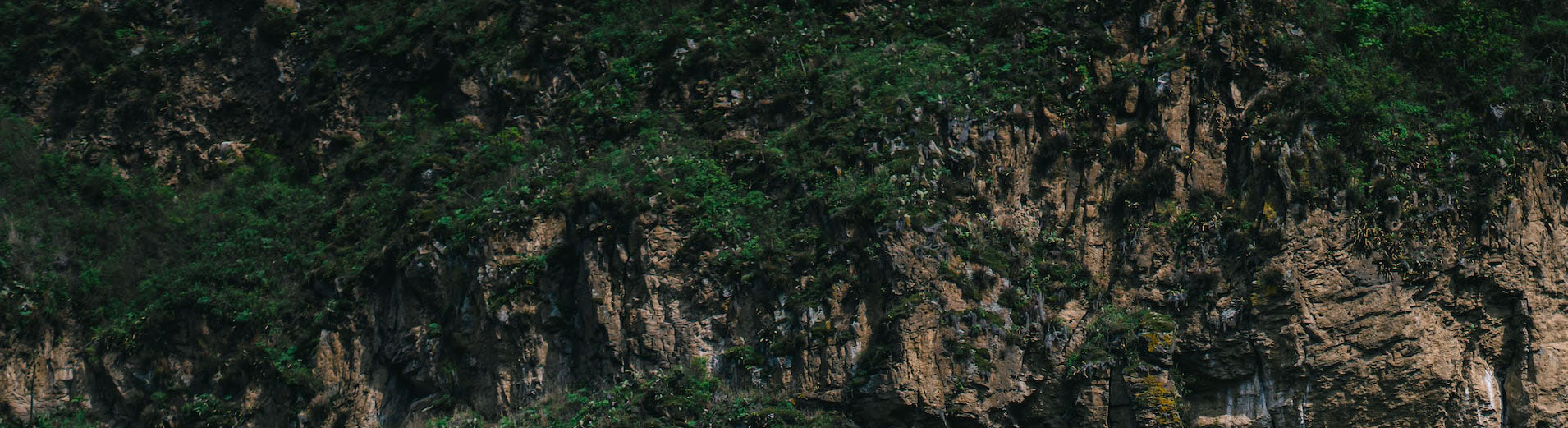
Downloads
Authors
Abstract
Providing accurate estimates of uncertainty is key for the analysis, adoption, and interpretation of species distribution models. In this manuscript, through the analysis of data from an emblematic North American cryptid, I illustrate how Conformal Prediction allows fast and informative uncertainty quantification. I discuss how the conformal predictions can be used to gain more knowledge about the importance of variables in driving presences and absences, and how they help assess the importance of climatic novelty when doing future predictions.
DOI
https://doi.org/10.32942/X2CD1J
Subjects
Ecology and Evolutionary Biology, Life Sciences
Keywords
species distribution model, machine learning, Uncertainty quantification, conformal prediction
Dates
Published: 2024-11-12 00:40
Last Updated: 2024-11-12 05:40
License
CC BY Attribution 4.0 International
Additional Metadata
Language:
English
Conflict of interest statement:
None
Data and Code Availability Statement:
Data and code available at https://github.com/tpoisot/ConformalSDM
There are no comments or no comments have been made public for this article.