This is a Preprint and has not been peer reviewed. This is version 1 of this Preprint.
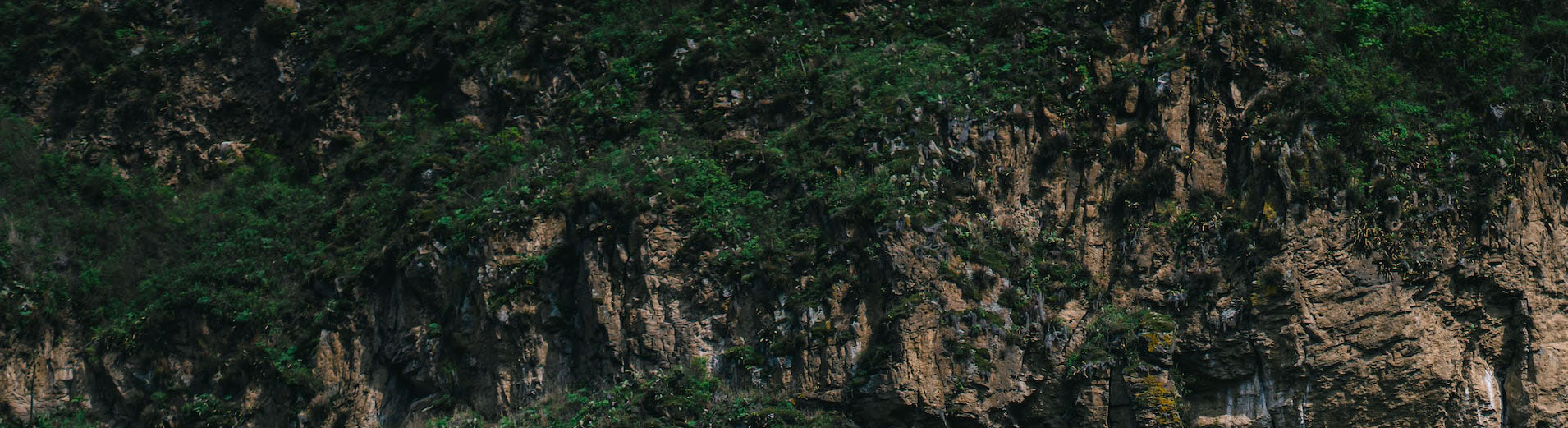
Offset or not: guidance on accounting for sampling effort in generalized linear models
Downloads
Authors
Abstract
1. Observed data are often dependent on a measure of sampling effort, such as counts measured per unit area. A common tool to account for differences in effort is the ‘offset term’ in a generalized linear model, which allows for a fixed proportional relationship between effort and the response variable. However, there is limited detailed guidance on the application of offsets and transformations or when an estimated effort covariate might be more appropriate.
2. This article explores the parametrisation and implementation of the offset term, plus additional methods to account for sampling effort in regression models. We evaluate the performance of offsets and covariates across various data characteristics through simulation.
3. When uncertainty regarding the effort–response relationship exists, modelling sampling effort as a log-transformed covariate, ideally as a constrained smoother, is ideal because it covers most scenarios: a proportional relationship, a non-linear (e.g. saturated) relationship, and flexibility in multi-species or hurdle models (e.g. allowing effort to influence detection probability in a binomial model). I show that parameter recovery in effort-as-covariate models is generally robust in simple models, so a log-transformed offset is only advantageous when: a proportional relationship is well-supported, model complexity or data availability hinders covariate estimation, or non-linearity at data limits is uncertain.
4. Although our simulation showed reasonable performance of all sampling effort parameterisations, how to model effort remains a key decision, and one that benefits from considered thought before modelling occurs. The nature of the effort–response relationship (i.e. proportional, otherwise linear on the link or original scales, or non-linear), and how multiple effort variables could be included in the same model, will benefit from both statistical and practical contexts and experience.
DOI
https://doi.org/10.32942/X2CP8Z
Subjects
Life Sciences
Keywords
GLMs, sampling effort, survey effort, offset, catch standardization, modelling
Dates
Published: 2024-10-09 02:52
License
CC-BY Attribution-NonCommercial 4.0 International
Additional Metadata
Conflict of interest statement:
None
Data and Code Availability Statement:
All code and data used in this study are available at: https://github.com/smithja16/Effort_Offset_Simulation
Language:
English
There are no comments or no comments have been made public for this article.