This is a Preprint and has not been peer reviewed. The published version of this Preprint is available: https://doi.org/10.1109/ehb64556.2024.10805656. This is version 2 of this Preprint.
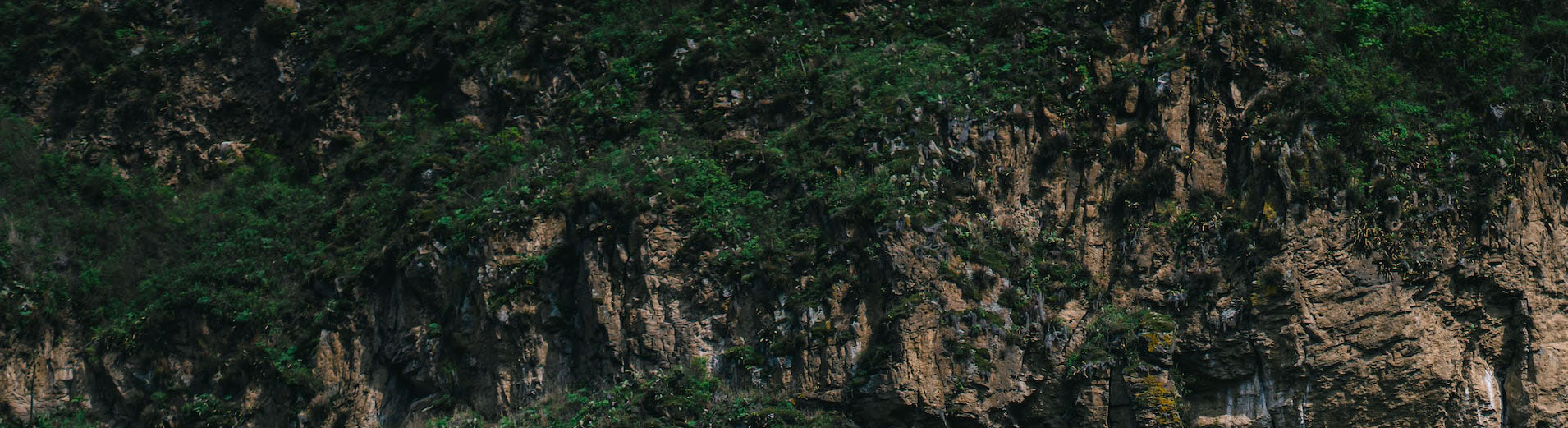
Leveraging ResNet-50 for Precision Toxicity Classification in Plants: A Vision-Based Approach to Safeguard Public Health
Downloads
Authors
Abstract
The classification of toxic and non-toxic plants plays an important role in ensuring public safety, especially in agriculture, food safety, and health. Correct identification of these plants can prevent accidental poisoning and promote ecological protection. In this paper, we investigate the application of the ResNet-50 model for the classification of toxic and non-toxic plants. Leveraging the powerful feature extraction techniques of the ResNet-50 architecture, the model achieved 89.6% accuracy, 87.4% precision, 91.1% recall, and an 89.2% F1 score, demonstrating the model’s effectiveness. Transfer learning proved effec-tive with limited data while maintaining high performance metrics in the classification task. Future research could focus on expanding the dataset to include more plant species and exploring other state-of-the-art models to improve classification accuracy. Addition-ally, integrating these models with mobile applications or monitoring systems could pro-vide solutions for business and public use, enhancing environmental protection and pub-lic safety.
DOI
https://doi.org/10.32942/X28W4K
Subjects
Environmental Engineering, Plant Sciences
Keywords
Poisonous plants, Non-poisonous plants, ResNet-50, Plant classification, Deep learning, transfer learning, accuracy
Dates
Published: 2024-10-04 03:25
Last Updated: 2025-02-06 23:56
Older Versions
License
CC BY Attribution 4.0 International
Additional Metadata
Language:
English
Data and Code Availability Statement:
Available on Kaggle
There are no comments or no comments have been made public for this article.