This is a Preprint and has not been peer reviewed. This is version 1 of this Preprint.
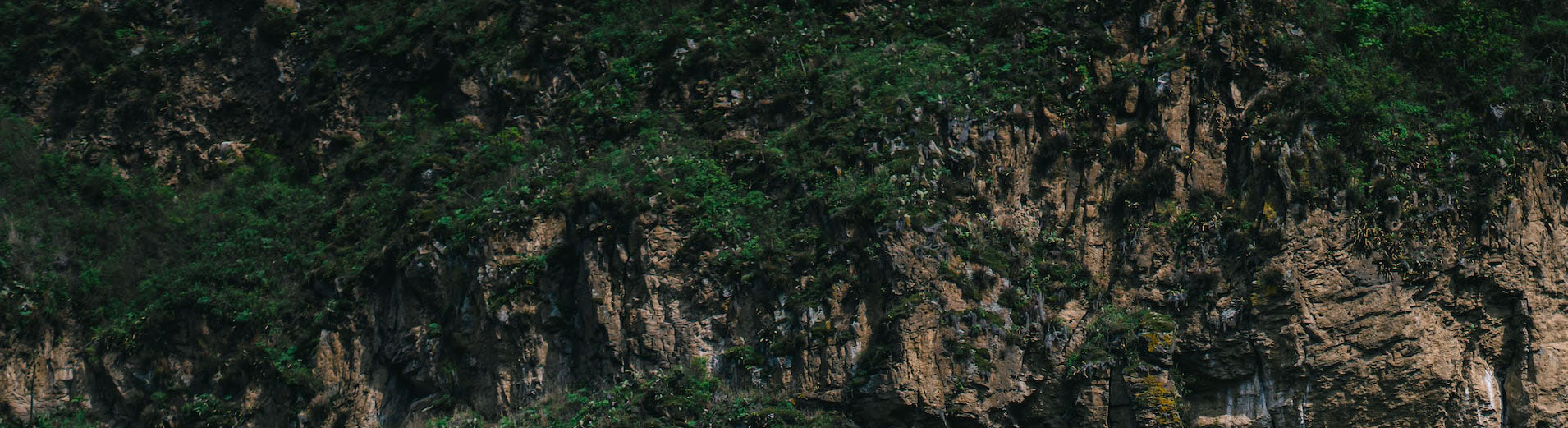
Copy-paste augmentation improves automatic species identification in camera trap images
Downloads
Authors
Abstract
1. Effective conservation requires effective biodiversity monitoring. The pace of global biodiversity change far outstrips the ability of manual fieldwork to monitor it. Therefore, technological solutions, like camera traps, have emerged as a crucial way to meet biodiversity monitoring needs. Camera traps produce vast amounts of data and so AI is increasingly used to label images with species identities. However, AI struggles to identify species from new locations that are not part of the training data (‘generalisation’). Resolving this is crucial for the promise of automated biodiversity monitoring to be realised.
2. Here we use ‘copy-paste’ augmentation to help resolve the generali- sation challenge. Copy-paste augmentation refers to isolating animal ‘segments’ from existing images and pasting the segments onto novel backgrounds, to create new, synthetic images that are then used as part of the training data. Theoretically, this could make a model agnostic to backgrounds and therefore more able to generalise to unseen locations. While generation of synthetic images is commonly used as an augmentation method in other fields, such as medicine, it has not been used before in biodiversity science.
3. We found that copy-paste augmentation improved the ability of AI to identify species in new, unseen locations by 8 ± 2%. There was species-level variation in improvement, but the vast majority of species benefited from the approach. We found mixed results when using copy-paste augmentation on models trained with very small numbers of images (1-8 per species).
4. Copy-paste augmentation improves the ability of AI models to gen- eralise to new, unseen locations. Our method also shows promise for resolving the challenge of long-tailed camera trap data. AIs perform poorly on species in the ‘long tail’ of these distributions because there are very few images to train on. Copy-paste augmentation can help rebalance datasets by adding synthetic images of underrep- resented species. Overall, our results suggest a promising role for augmentation methods that generate new, synthetic images in bio- diversity science. Ecologists and conservationists must move beyond simple augmentation methods, such as image transformations, if we are to resolve key challenges in species identification AI.
DOI
https://doi.org/10.32942/X2VS6K
Subjects
Biodiversity, Ecology and Evolutionary Biology
Keywords
camera trap, AI, machine learning, augmentation, computer vision, Mammals, serengeti, monitoring
Dates
Published: 2024-09-24 21:38
License
CC BY Attribution 4.0 International
Additional Metadata
Language:
English
Data and Code Availability Statement:
The Serengeti dataset is publicly accessible at https://lila.science/datasets/snapshot-serengeti. Our code is available at https://doi.org/10.5281/zenodo.12773166.
There are no comments or no comments have been made public for this article.