This is a Preprint and has not been peer reviewed. This is version 1 of this Preprint.
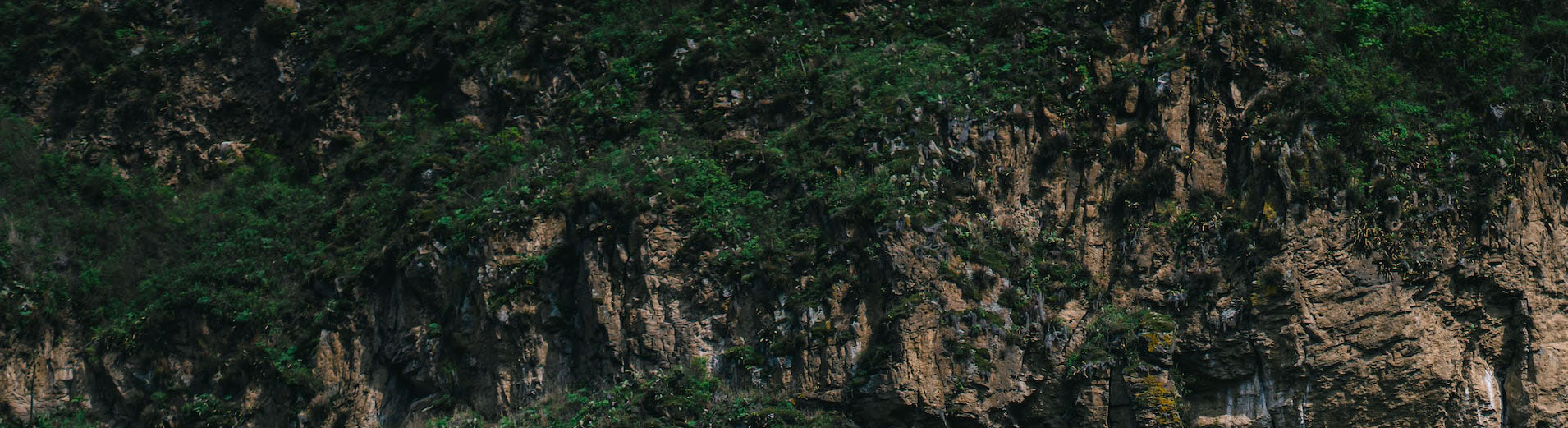
Developing Machine Learning Applications for Population Genetic Inference: Ensuring Precise Terminology and Robust Implementation
Downloads
Authors
Abstract
Machine learning applications for population genetic inference are emerging due to their potential to leverage large-scale genomic datasets, offering insights that traditional statistical methods may overlook. However, I have identified certain recurring issues. First, there is sometimes confusion between power and recall, and between the false discovery rate and one minus precision. These terms are specifically designed for hypothesis testing and are not appropriate for directly evaluating classification outcomes, as classification is a different task. Second, the lack of robustness in machine learning applications complicates their verification and application across different datasets, limiting their broader impact and slowing research progress. Robustness can be improved through strategies such as employing object-oriented programming for design, utilizing version control systems during development, and adopting package managers and workflow managers for distribution. I suggest by adhering to precise terminology and refining implementation practices, the impact of machine learning in population genetics can be maximized.
DOI
https://doi.org/10.32942/X2N90M
Subjects
Life Sciences
Keywords
Dates
Published: 2024-09-20 21:14
License
CC-BY Attribution-NonCommercial-ShareAlike 4.0 International
Additional Metadata
Language:
English
There are no comments or no comments have been made public for this article.