This is a Preprint and has not been peer reviewed. This is version 1 of this Preprint.
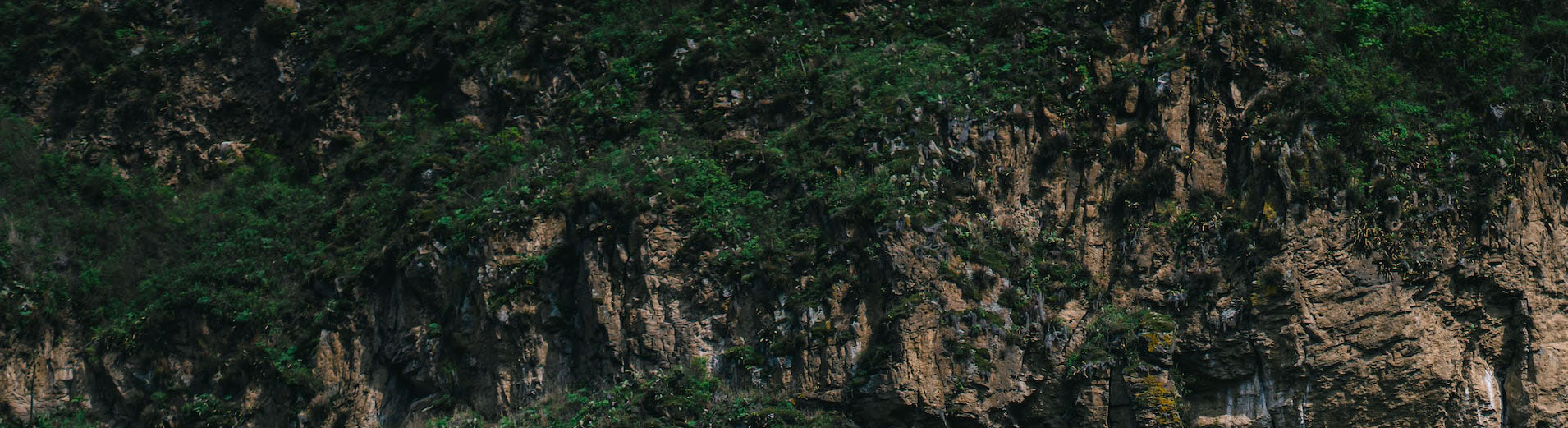
Evaluating drivers and predictability of catch composition in a highly mixed trawl fishery using stacked and joint species models
Downloads
Supplementary Files
Authors
Abstract
Evaluating drivers and the predictability of catch is valuable for the management of mixed fisheries. Drivers can represent or help to identify levers for management and predictable catch compositions are a key component of simulation tools and dynamic management strategies. But modelling mixed fisheries can be challenging due to the large number of taxa, and analysis typically focuses on a few key species or highly aggregated taxa.
Here we employ seven types of stacked and joint species models to explore the drivers and predictability of trawl-level catches in an ocean prawn trawl fishery, in New South Wales, Australia. Catch data was sourced from an observer program, with 130 taxa able to be modelled. The main drivers of catch composition were latitude, depth, and seasonality represented here by water temperature. Water column mixing, lunar illumination, and fishing effort were also important for some taxa. Up to 60-80 taxa had good predictive skill (AUC>0.8, >35% decline in mean absolute error relative to an intercept-only model), and an additional 40-60 taxa had lower but still useful predictive skill (AUC>0.7, 25-35% decline in error). However, the number of skilful taxa varied considerably among model type.
The best framework for prediction was stacked random forests using a hurdle modelling approach, followed by a spatial joint species model. Our results show that predictive models at a fine spatial-temporal and taxonomic resolutions can be a viable information tool for highly mixed fisheries, but these tools ultimately need to be tested against specific management objectives and performance metrics, such as spatial closures and bycatch:target catch ratios.
DOI
https://doi.org/10.32942/X2W33V
Subjects
Life Sciences
Keywords
species distribution modelling, SDM, bycatch, penaeid, spatial management, bycatch, spatial management
Dates
Published: 2024-08-13 20:44
Last Updated: 2024-08-14 00:44
License
CC-BY Attribution-NonCommercial 4.0 International
Additional Metadata
Conflict of interest statement:
None
Data and Code Availability Statement:
The observer data used in this analysis is confidential and cannot be shared. To access an aggregated and anonymised version contact the authors. R code for this study is available at https://github.com/smithja16/MultiSpeciesModels_Observer.
Language:
English
There are no comments or no comments have been made public for this article.