This is a Preprint and has not been peer reviewed. This is version 2 of this Preprint.
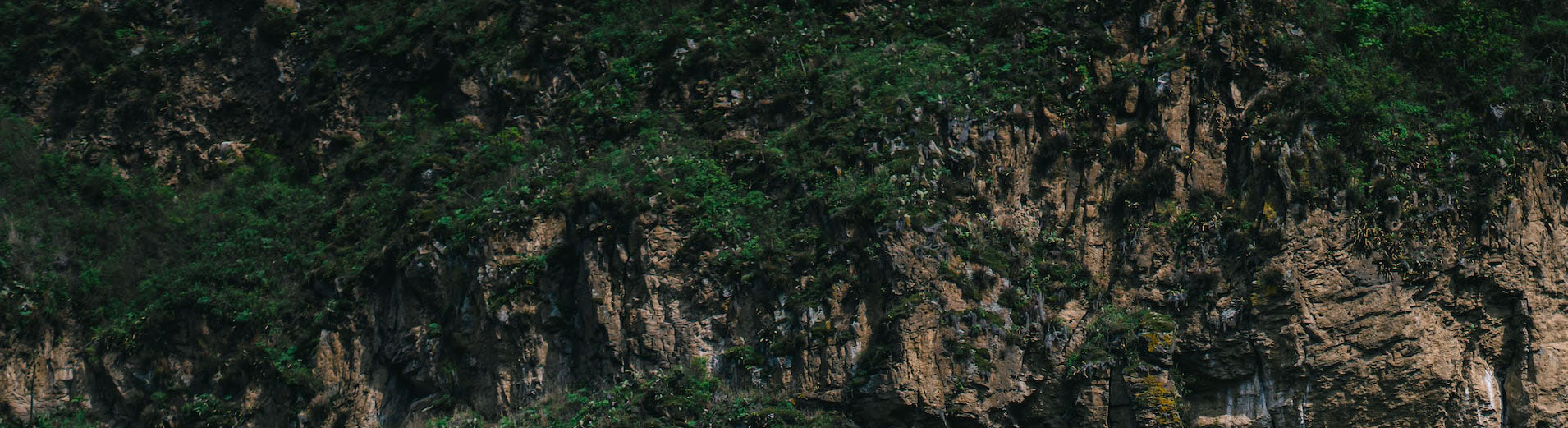
The Architecture of Theory and Data in Microbiome Design: towards an S-matrix for microbiomes
Downloads
Authors
Abstract
Designing microbiomes for applications in health, bioengineering, and sustainability is intrinsically linked to a fundamental theoretical understanding of the rules governing microbial community assembly. Microbial ecologists have used a range of mathematical models to understand, predict, and control microbiomes, ranging from mechanistic models, putting microbial populations and their interactions as the focus, to purely statistical approaches, searching for patterns in empirical and experimental data. We review the success and limitations of these modeling approaches when designing novel microbiomes, especially when guided by (inevitably) incomplete experimental data. Although successful at predicting generic patterns of community assembly, mechanistic and phenomenological models tend to fall short of the precision needed to design and implement specific functionality in a microbiome. We argue that to effectively design microbiomes with optimal functions in diverse environments, ecologists should combine data-driven techniques with mechanistic models---a middle, third way for using theory to inform design.
DOI
https://doi.org/10.32942/X2SD0W
Subjects
Ecology and Evolutionary Biology, Life Sciences
Keywords
microbiome design, microbial ecology
Dates
Published: 2024-08-09 14:25
Last Updated: 2024-12-22 02:55
Older Versions
License
CC-BY Attribution-NonCommercial 4.0 International
Additional Metadata
Language:
English
There are no comments or no comments have been made public for this article.