This is a Preprint and has not been peer reviewed. The published version of this Preprint is available: https://doi.org/10.1038/s41559-025-02715-6. This is version 7 of this Preprint.
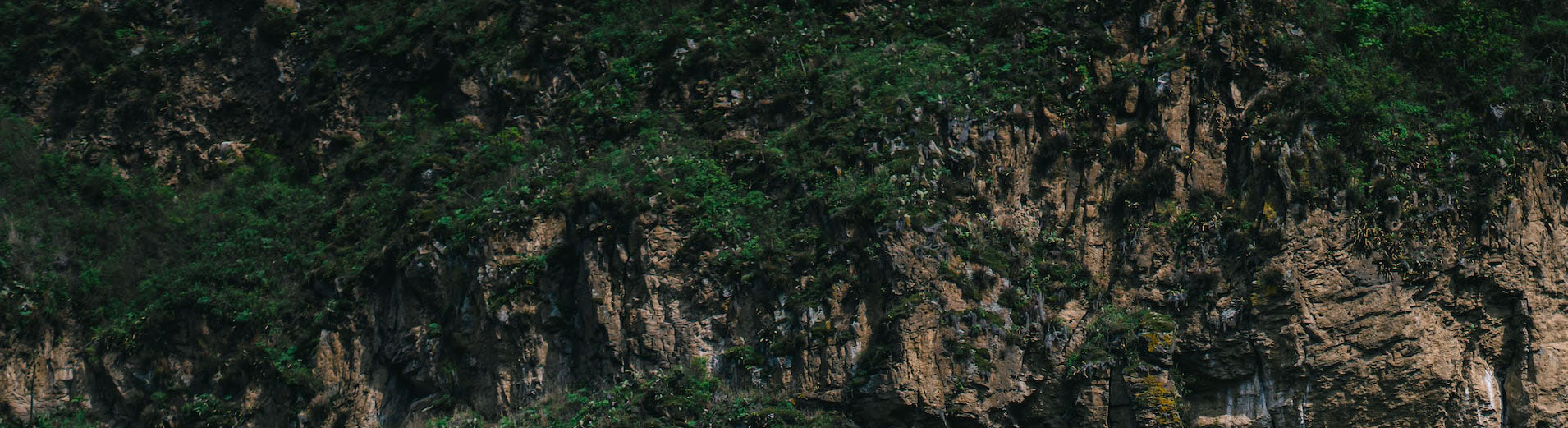
Inductive link prediction facilitates the discovery of missing links and enables cross-community inference in ecological networks
Downloads
Authors
Abstract
Predicting species interactions (links) within ecological networks is crucial for advancing our understanding of ecosystem functioning and responses of communities to environmental changes. Transductive link prediction models are often used but are constrained by sparse, incomplete data and are limited to single networks. We address these issues using an inductive link prediction (ILP) approach. We evaluated the model performance on 538 networks across four community types: plant-seed disperser, plant-pollinator, host-parasite, and plant-herbivore. By pooling data across communities and applying transfer learning, our model predicts interactions within and between ecological networks. The ILP model achieved higher precision and F1 scores than transductive models. However, cross-community prediction efficacy varies, with plant-seed disperser and host-parasite networks performing better than plant-pollinator and plant-herbivore networks as training and test sets. Moreover, the nature of ecological interactions (mutualistic versus antagonistic) did not strongly influence the predictability of missing links. Finally, leveraging ILP’s generalizability, we developed a pre-trained model that ecologists can readily use to make instant predictions for their networks. This study highlights ILP’s potential to improve the prediction of ecological interactions, enabling generalization across diverse ecological contexts and bridging critical data gaps.
DOI
https://doi.org/10.32942/X2JS75
Subjects
Ecology and Evolutionary Biology
Keywords
link prediction, machine learning, transfer learning, ecological networks
Dates
Published: 2024-08-02 21:27
Last Updated: 2025-06-05 05:25
Older Versions
- Version 6 - 2025-05-12
- Version 5 - 2025-05-12
- Version 4 - 2025-05-12
- Version 3 - 2025-04-24
- Version 2 - 2025-02-07
- Version 1 - 2024-08-02
License
CC-BY Attribution-NonCommercial-ShareAlike 4.0 International
Additional Metadata
Language:
English
Data and Code Availability Statement:
The data are available in the repository set up in original publication https://osf.io/my9tv/. The full code and technical descriptions on how to run the pipeline are available on the GitHub repository https://github.com/Ecological-Complexity-Lab/eco_ILP
There are no comments or no comments have been made public for this article.