This is a Preprint and has not been peer reviewed. This is version 1 of this Preprint.
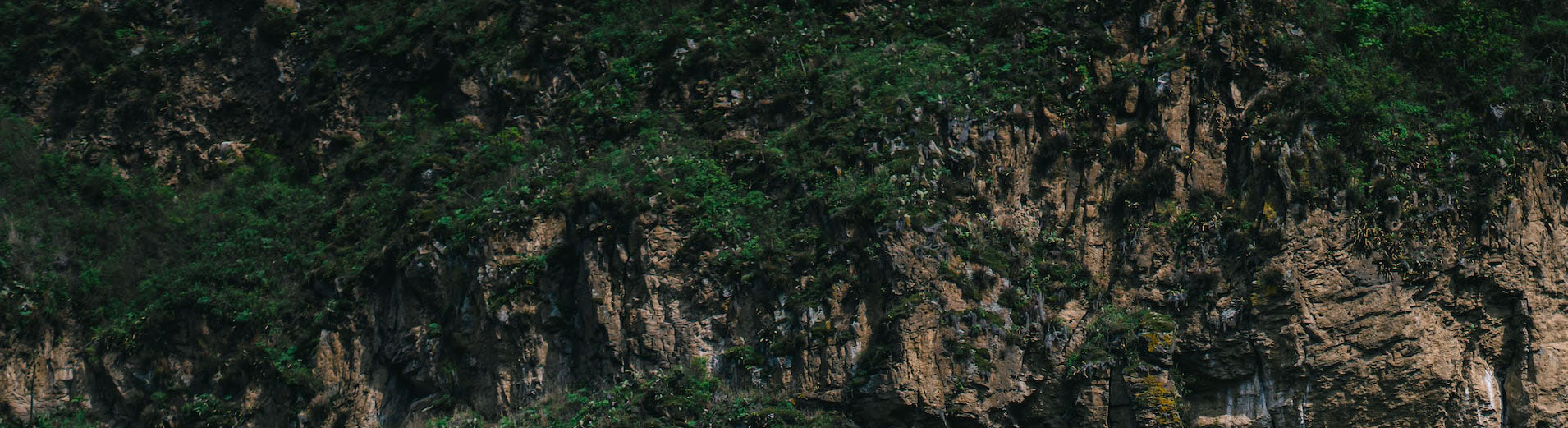
Making use of spatially biased variables in ecosystem condition accounting – a GIS based workflow
Downloads
Supplementary Files
Authors
Abstract
Ecosystem Condition Accounts (ECA) should reflect the integrity or quality of all nature inside the scope of the account, and therefore rely on spatially representative indicators for condition. All ECAs are subject to data constraints in some way. Therefore, being able to make use of spatially biased data sets would be very valuable. For national ECAs, modelling approaches can in some cases be used to control for sampling biases. For local ECAs however, like at the scale of individual municipalities of development projects, this is often not an option as it involves spatial extrapolation using data from outside the ecosystem accounting area. In this study we develop three ecosystem condition indicators from the same spatially biased data set based on nature type mapping of Norwegian mires. The indicators are Alien species, Trenching and Anthropogenic Disturbance to Soil and Vegetation. We test our approach in three municipalities in south Norway. We discretised a spatial variable representing infrastructure prevalence, and we refer to this new map as Homogeneous Impact Areas (HIAs). To facilitate reliable estimation of indicator uncertainties, even in cases with very low sample sizes, we use a Bayesian updating method and produce probability distributions for the area-weighted mean indicator values in each HIA separately. Then we use area-weighted resampling and produce indicator probability distributions for each municipality. With this Bayesian updating and resampling approach, small sample sizes can be compensated by correspondingly large uncertainty ranges, as long as the full dataset is large enough to estimate the true population standard deviation. This paper demonstrates the use of a GIS-based workflow to control for some of the most problematic biases in an opportunistic field survey so that the data can be used for indicators in ECAs. The workflow can be used at any scale, including national scale. Because indicator values are calculated for unique spatial strata, local governments or others can target their data acquisition towards strata with low sample sizes, and thus achieve higher cost effectiveness and ultimately better spatial indicator coverage.
DOI
https://doi.org/10.32942/X2K900
Subjects
Ecology and Evolutionary Biology, Environmental Indicators and Impact Assessment, Environmental Monitoring
Keywords
alien species, disturbance, ecosystem accounting, Ecosystem Condition, Horizontal Aggregation, Indicator, Mire, peatland, SEEA EA, wetland
Dates
Published: 2024-06-06 13:40
License
CC BY Attribution 4.0 International
Additional Metadata
Conflict of interest statement:
None
Data and Code Availability Statement:
This manuscript is written in Quarto, and the source file (Appendix B) also contains code for all the analyses underlying this study, including data exploration and cleaning. For a rendered version of the source file, with all code and calculation visible, see Appendix C. The source files and the data are also located on GitHub (https://github.com/anders-kolstad/HIAs) with an archived stable version on Zenodo (doi:10.5281/zenodo.11504703). The exception is the large nature type survey data, which was downloaded locally, but which is freely available.
Language:
English
There are no comments or no comments have been made public for this article.