This is a Preprint and has not been peer reviewed. This is version 1 of this Preprint.
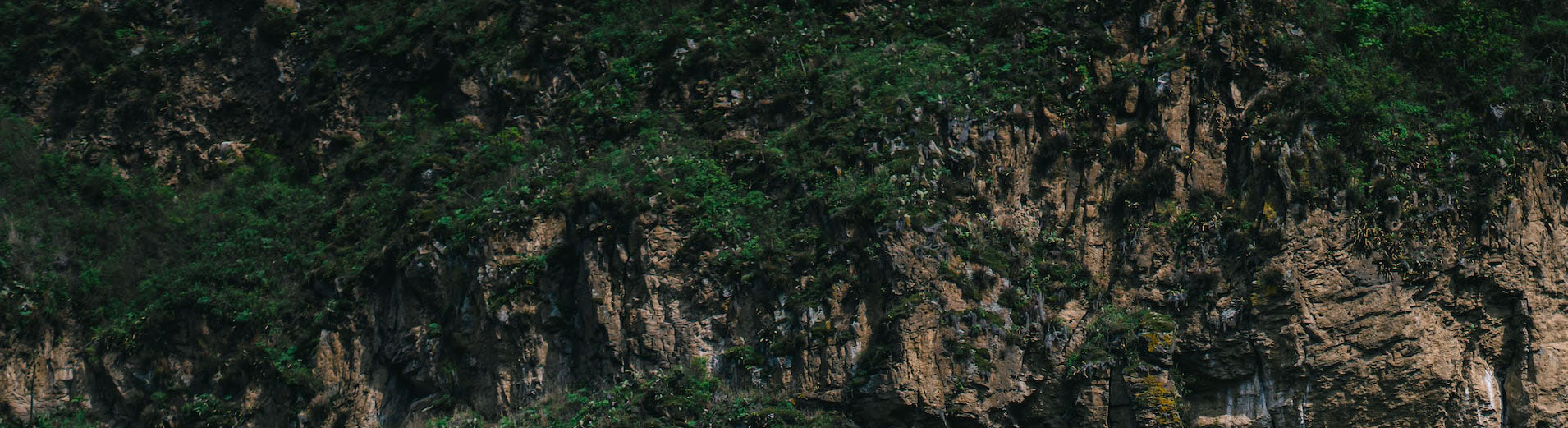
Downloads
Authors
Abstract
Accurate estimates of abundance are crucial for successful conservation and management. However, gathering abundance data is costly. Species Abundance Models (SAMs) are increasingly used to predict variation in abundance for resource management for single species, but collecting enough relevant environmental information to build effective SAMs can often be challenging. Species co-occurrence patterns may provide additional information on missing environmental predictors, and data on presence-absence species co-occurrence are typically easier to collect than abundance or detailed environmental data. However, it is still not clear when supplementing abiotic data with co-occurrence data should improve abundance predictions, as co-occurrence data itself represents a noisy indicator of the local environment. Using simulated data where we manipulated the strength of relevant environmental predictors across multiple species, we assessed the conditions that improve model predictions of a target species by using co-occurrence data on the remaining species as a proxy for missing environmental predictors. Because species often share environmental preferences in nature, an aspect simulated in our data, latent variables are expected to summarize important environmental gradients across co-occurring species. We employed Gaussian copulas to generate presence-absence co-occurrence-based latent variables as proxies. These latent variables, along with various combinations of environmental predictors, were subsequently used as predictors in SAMs. We evaluated the accuracy of these models in predicting the presence and abundance of target species through model validation exercises. Our results showed that incorporating presence-absence latent predictors generally improved model performance when compared to models lacking relevant environmental predictors, although there was considerable variation in performance across simulations. All models tended to have greater error rates when predicting abundant species compared to rare species. The goal of our proposed framework is to offer a novel and easy to implement method for accurately predicting abundance from both biotic and environmental information.
DOI
https://doi.org/10.32942/X2S32J
Subjects
Aquaculture and Fisheries Life Sciences, Biology
Keywords
abundance, co-distribution, co-occurrence, latent, prediction, Simulation
Dates
Published: 2024-05-10 20:36
License
CC-BY Attribution-NonCommercial-ShareAlike 4.0 International
Additional Metadata
Language:
English
Conflict of interest statement:
None
Data and Code Availability Statement:
https://doi.org/10.5281/zenodo.11150229
There are no comments or no comments have been made public for this article.