This is a Preprint and has not been peer reviewed. This is version 1 of this Preprint.
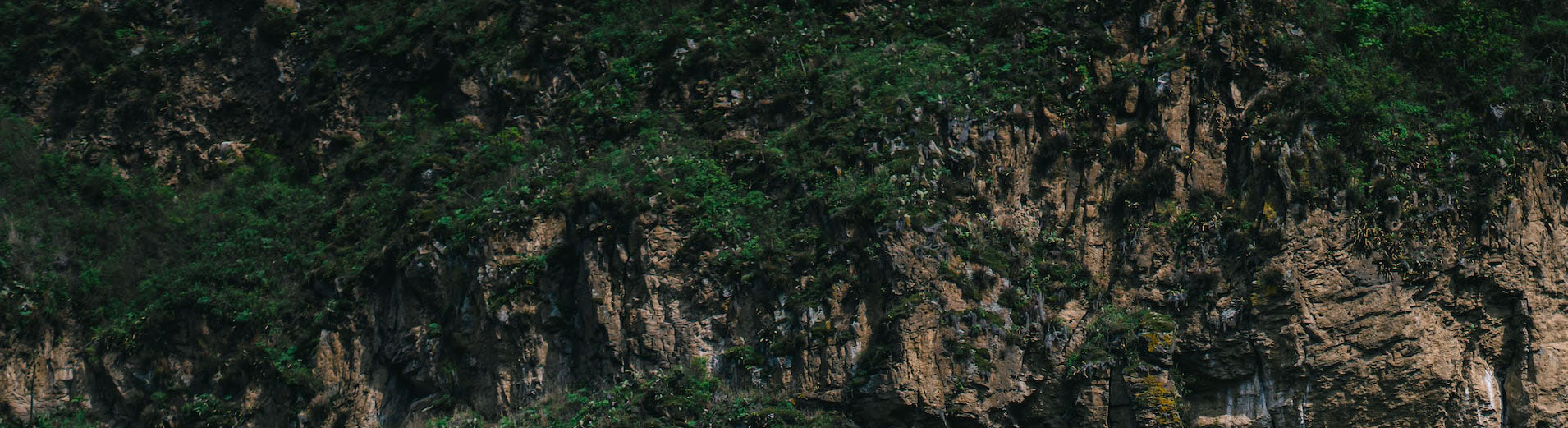
Downloads
Authors
Abstract
Just like data, models have their own life cycle. By recognizing how one’s model fits within the life cycle of the data (or at least, ensuring that the model life cycle is understood), we can identify opportunities to foster new collaborations, encourage better practices in data analysis, and ultimately accelerate research. In this manuscript, we introduce the Model Life Cycle and develop a series of ten simple rules aimed at facilitating collaborations between data collectors, curators, users, and modellers, as well as maximizing the potential for re-use of models. We explore the idea of a Model Life Cycle, starting from the assumption that it will address machine learning (ML) models, i.e. models that can be trained and deployed iteratively, and whose focus is on prediction of quantifiable phenomena. Specifically, we are interested in clarifying the use of models in large, interdisciplinary groups, where the actual modelling exercise may involve only a subset of the group (e.g., with others collecting and standardizing data).
DOI
https://doi.org/10.32942/X2XG8V
Subjects
Bioinformatics, Ecology and Evolutionary Biology, Software Engineering
Keywords
model life cycle, data life cycle, Iterative forecasting
Dates
Published: 2024-02-10 03:58
Last Updated: 2024-02-10 08:58
License
CC BY Attribution 4.0 International
Additional Metadata
Language:
English
Data and Code Availability Statement:
Not applicable
There are no comments or no comments have been made public for this article.