This is a Preprint and has not been peer reviewed. This is version 1 of this Preprint.
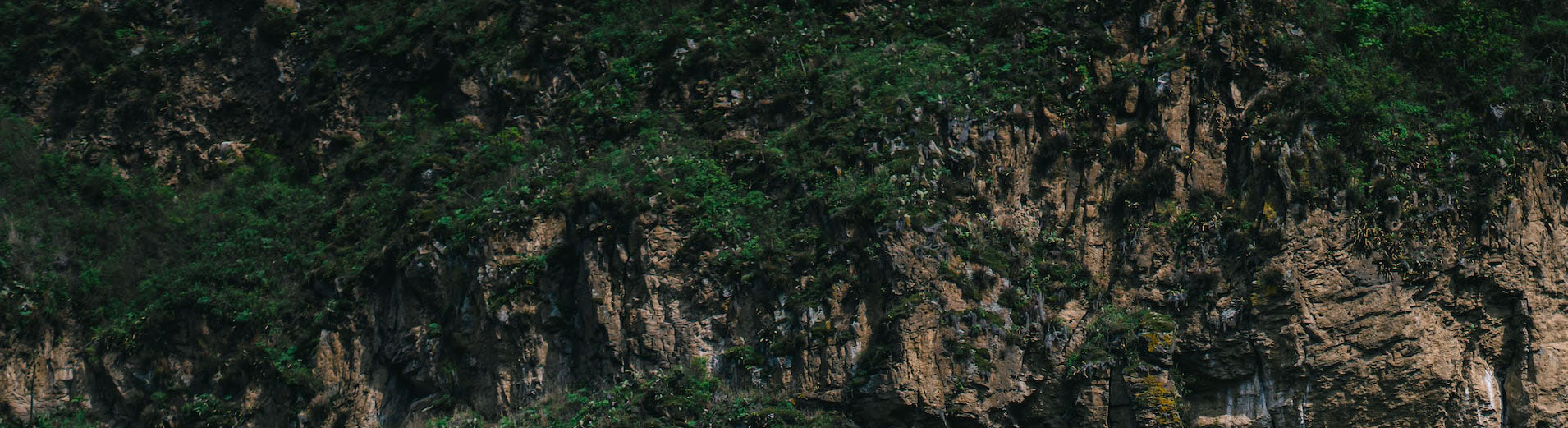
Downloads
Authors
Abstract
Estimating underlying cooccurrence relationships between pairs of species has long been a challenging task in ecology as the extent to which species actually cooccur is partially dependent on their prevalences. While recent work has taken large steps towards solving this problem, the next question is how to assess the factors that influence cooccurrence. Here I show that a recently proposed cooccurrence metric can be improved upon by assigning Bayesian priors to the latent cooccurrence relationships being estimated. In the context of analysing the factors that affect cooccurrence relationships, I demonstrate the need for a generalised linear model (GLM) that takes cooccurrences and species prevalence (not cooccurrence metrics) as its data. Finally, I show the form that such a GLM should take in order to perform Bayesian inference while accounting for non-independence of dyadic matrix data (e.g. distance and cooccurrence matrices).
DOI
https://doi.org/10.32942/X2KC8Q
Subjects
Life Sciences
Keywords
Bayesian, ecology, cooccurrence
Dates
Published: 2024-01-23 19:57
License
CC-By Attribution-NonCommercial-NoDerivatives 4.0 International
Additional Metadata
Language:
English
Data and Code Availability Statement:
All code to produce the figures and the manuscript can be found at https://github.com/EvoArt/bayesian-affinity.
There are no comments or no comments have been made public for this article.