This is a Preprint and has not been peer reviewed. This is version 1 of this Preprint.
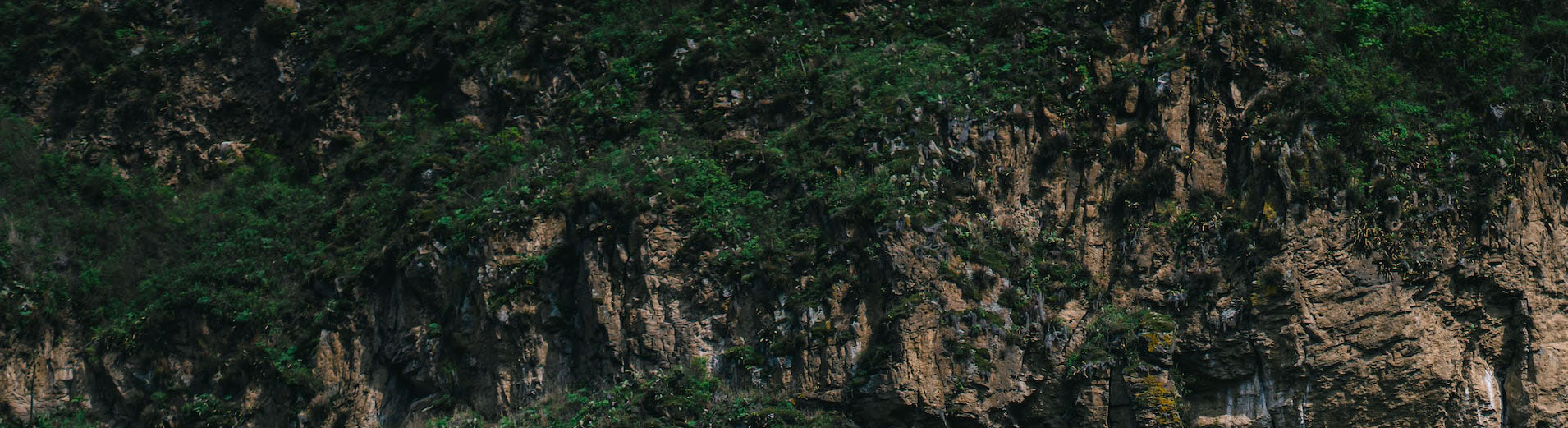
Inferring the seasonal dynamics and abundance of an invasive species using a spatio-temporal stacked machine learning model
Downloads
Authors
Abstract
Various modelling techniques are available to understand the temporal and spatial variations of the phenology of species. Scientists often rely on correlative models, which establish a statistical relationship between a response variable (such as species abundance or presence-absence) and a set of predominantly abiotic covariates. The modelling approach choice, i.e. the algorithm, is a crucial factor for addressing the multiple sources of variability that can lead to disparate outcomes when different models are applied to the same dataset. This inter-model variability has led to the adoption of ensemble modelling techniques, among which stacked generalisation, which has recently demonstrated its capacity to produce robust results. Stacked ensemble modelling incorporates predictions from multiple base learners or models as inputs for a meta-learner. The meta-learner, in turn, assimilates these predictions and generates a final prediction by combining the information from all the base learners. In our study, we utilized a recently published dataset documenting egg abundance observations of Aedes albopictus collected using ovitraps. This dataset spans various locations in southern Europe, covering four countries - Albania, France, Italy, and Switzerland - and encompasses multiple seasons from 2010 to 2022. Utilising these ovitrap observations and a set of environmental predictors, we employed a stacked machine learning model to forecast the weekly average number of mosquito eggs. This approach enabled us to i) unearth the seasonal dynamics of Ae. albopictus for 12 years; ii) generate spatio-temporal explicit forecasts of mosquito egg abundance in regions not covered by conventional monitoring initiatives. Beyond its immediate application for public health management, our work presents a versatile modelling framework adaptable to infer the spatio-temporal abundance of various species, extending its relevance beyond the specific case of Ae. albopictus.
DOI
https://doi.org/10.32942/X2NG70
Subjects
Biology, Entomology, Life Sciences, Population Biology, Public Health, Virus Diseases
Keywords
arthropod, Aedes albopictus, Forecast, invasive species, population dynamics, Time-series
Dates
Published: 2023-12-22 06:54
License
CC BY Attribution 4.0 International
Additional Metadata
Conflict of interest statement:
None
Data and Code Availability Statement:
the link to the repository hosting the scripts used is available in the preprint
Language:
English
There are no comments or no comments have been made public for this article.