This is a Preprint and has not been peer reviewed. This is version 2 of this Preprint.
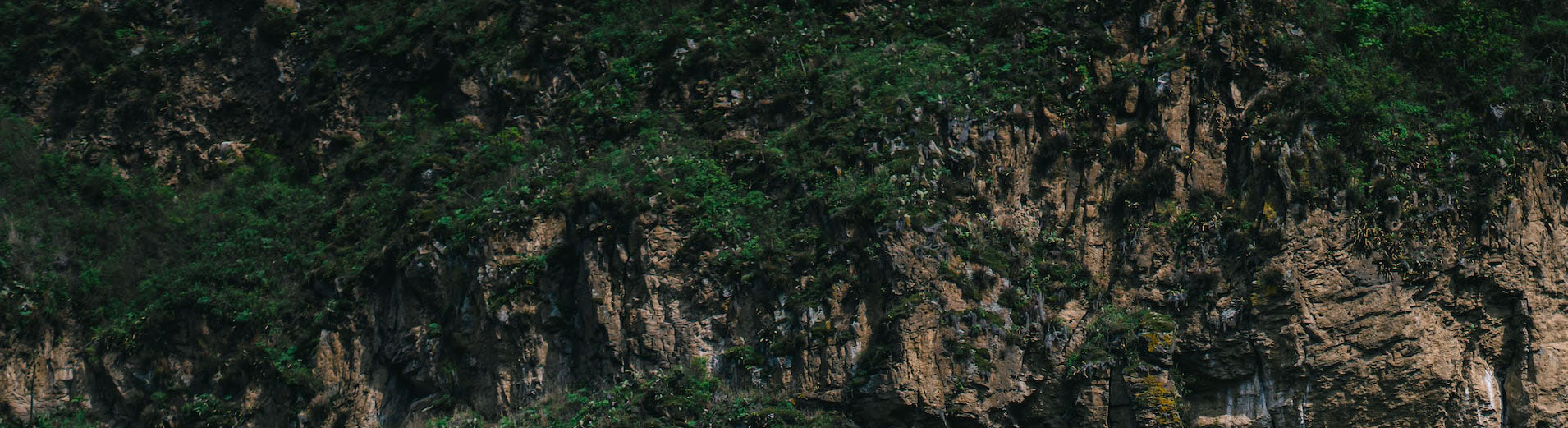
Downloads
Authors
Abstract
Monitoring wildlife is crucial for making informed conservation and land-management decisions. Remotely triggered cameras are widely used for this purpose, but the resulting 'big data' are laborious to process. Although artificial intelligence (AI) offers a powerful solution to this bottleneck, it has been challenging for ecologists and practitioners without substantial technical expertise to tailor current approaches to their specific use cases. Generic, online offerings also have issues of ongoing costs and data privacy. Here we present an open-source, scalable, modular, cross-platform workflow, deployed using Docker containers, which leverages deep learning for wildlife-image classification. It can be run using simple command-line prompts or via a user-friendly Graphical User interface (EcoAssist). It enables end-users to easily execute a full range of tasks—from animal detection and counting to species identification—on local or cloud GPU-accelerated machines. It also integrates with the widely used open-source camera-trapping software ‘Camelot’, writing AI-classification data directly to image metadata and to CSV files, ready for either expert verification or direct data analysis. The result is a user-friendly but powerful multi-platform application for wildlife-image classification and research pipelines. An example case study with Tasmanian wildlife demonstrates the utility of our classifier training and inference workflow.
DOI
https://doi.org/10.32942/X2ZW3D
Subjects
Biodiversity, Ecology and Evolutionary Biology, Life Sciences, Zoology
Keywords
Wildlife monitoring, Artificial Intelligence, image classification, Deep learning, camera trapping, open-source software, Ecological Data Processing, docker, Camelot, MegaDetector
Dates
Published: 2023-12-12 00:32
Last Updated: 2024-09-18 05:31
Older Versions
License
CC-BY Attribution-NonCommercial 4.0 International
Additional Metadata
Language:
English
Conflict of interest statement:
None
Data and Code Availability Statement:
Code (open source): http://github.com/zaandahl/mewc; Data: Brook, B, Buettel, J (2023) Mega-Efficient Wildlife Classifier (MEWC) Case Study. https://dx.doi.org/10.25959/wm5g-b990
There are no comments or no comments have been made public for this article.