This is a Preprint and has not been peer reviewed. The published version of this Preprint is available: https://doi.org/10.32942/X2W313. This is version 4 of this Preprint.
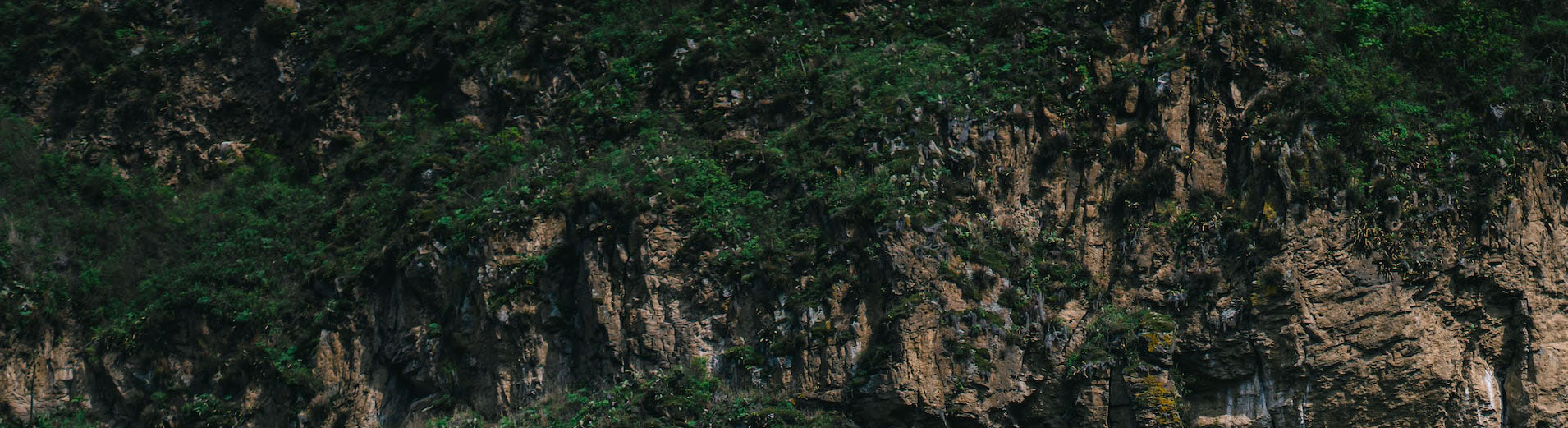
Towards the next generation of species delimitation methods: an overview of Machine Learning applications
Downloads
Supplementary Files
Authors
Abstract
Species delimitation is the process of distinguishing between populations of the same species and distinct species of a particular group of organisms. Various methods exist for inferring species limits, whether based on morphological, molecular, or other types of data. In the case of methods based on DNA sequences, most of them are rooted in the coalescent theory. However, coalescence-based models have limitations, for instance regarding complex evolutionary scenarios and large datasets. In this context, machine learning (ML) can be considered as a promising analytical tool, and provides an effective way to explore dataset structures when species-level divergences are hypothesized. In this review, we examine the use of ML in species delimitation and provide an overview and critical appraisal of existing workflows. We also provide simple explanations on how the main types of ML approaches operate, which should help uninitiated researchers and students interested in the field. Our review suggests that while current ML methods designed to infer species limits are analytically powerful, they also present specific limitations and should not be considered as definitive alternatives to coalescent methods for species delimitation. Future ML enterprises to delimit species should consider the constraints related to the use of simulated data, as in other model-based methods relying on simulations. Conversely, the flexibility of ML algorithms offers a significant advantage by enabling the analysis of diverse data types (e.g., genetic and phenotypic) and handling large datasets effectively. We also propose best practices for the use of ML methods in species delimitation, offering insights into potential future applications. We expect that the proposed guidelines will be useful for enhancing the accessibility, effectiveness, and objectivity of ML in species delimitation.
DOI
https://doi.org/10.32942/X2W313
Subjects
Biology, Computational Biology, Ecology and Evolutionary Biology, Genetics and Genomics
Keywords
Bioinformatics, molecular data, speciation, phylogenetics, phylogenomics, Artificial intelligence, deep learning., molecular data, speciation, phylogenetics, phylogenomics, Artificial Intelligence, Deep learning
Dates
Published: 2023-12-07 09:20
Last Updated: 2025-03-03 13:43
Older Versions
License
CC BY Attribution 4.0 International
Additional Metadata
Language:
English
Data and Code Availability Statement:
Not applicable
There are no comments or no comments have been made public for this article.