This is a Preprint and has not been peer reviewed. This is version 4 of this Preprint.
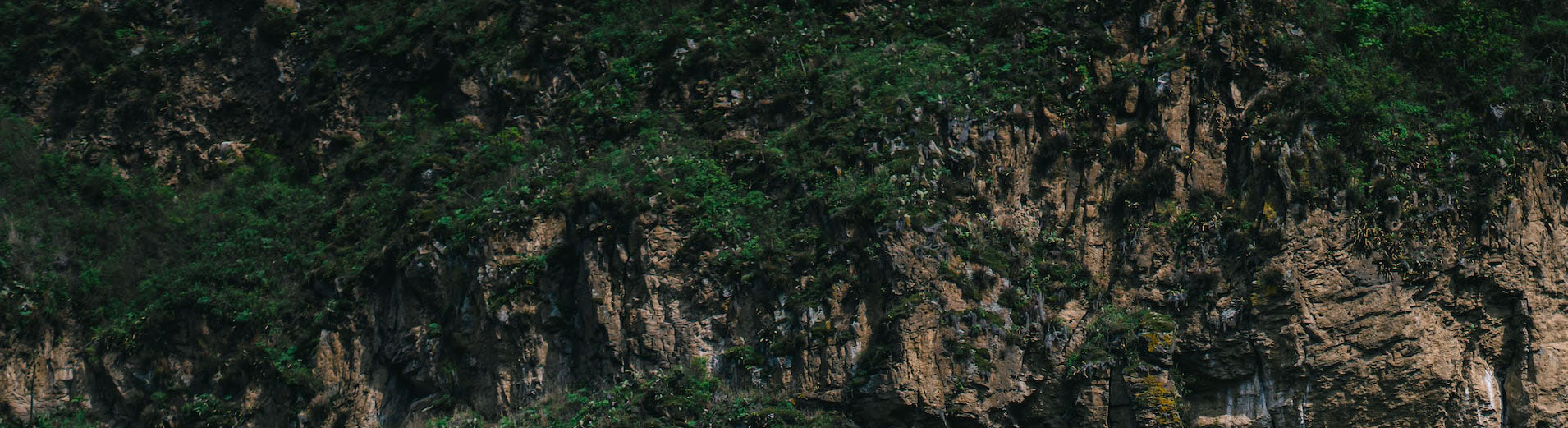
Treating gaps and biases in biodiversity data as a missing data problem
Downloads
Authors
Abstract
Big biodiversity datasets have great potential for monitoring and research because of their large taxonomic, geographic and temporal scope. Such datasets have become especially important for assessing the temporal change of species’ populations and distributions. Gaps in the available data, however, often hinder drawing large-scale inferences about species’ trends. Here, we conceptualise biodiversity data gaps as a missing data problem, which provides a unifying framework for the challenges and potential solutions across different types of biodiversity datasets. We characterise the typical types of data gaps in biodiversity data as different classes of missing data and then use missing data theory to explore the implications for different research questions. By using this framework, we show that bias due to data gaps can arise when the factors affecting sampling and/or data availability overlap with those affecting biodiversity. But the outcome also depends on the ecological questions, which determines choices around the analytical approach. We argue that typical approaches to long-term species trend modelling are especially susceptible to data gaps since such models do not tend to account for the factors that drive missingness. To identify general solutions, we review empirical studies and use simulation studies to compare some of the most frequently employed approaches to deal with data gaps, including subsampling, weighting and imputation. All these methods have the potential to reduce bias but may come at the cost of increased uncertainty of parameter estimates. Weighting approaches are arguably the least used so far in ecology and have the potential to reduce both the bias and variance of parameter estimates. Regardless of the method, the ability to reduce bias critically depends on knowledge of, and the availability of data on, the factors creating data gaps. We use our review to outline the necessary considerations when dealing with data gaps at different stages of the data collection and analysis workflow.
DOI
https://doi.org/10.32942/X2089S
Subjects
Life Sciences
Keywords
biodiversity change, big data, citizen science, Trend modelling, monitoring, macroecology, Spatial bias
Dates
Published: 2023-10-22 06:54
Last Updated: 2023-10-22 10:54
Older Versions
License
CC BY Attribution 4.0 International
Additional Metadata
Language:
English
Data and Code Availability Statement:
Not applicable
There are no comments or no comments have been made public for this article.