This is a Preprint and has not been peer reviewed. The published version of this Preprint is available: https://doi.org/10.1371/journal.pclm.0000290. This is version 3 of this Preprint.
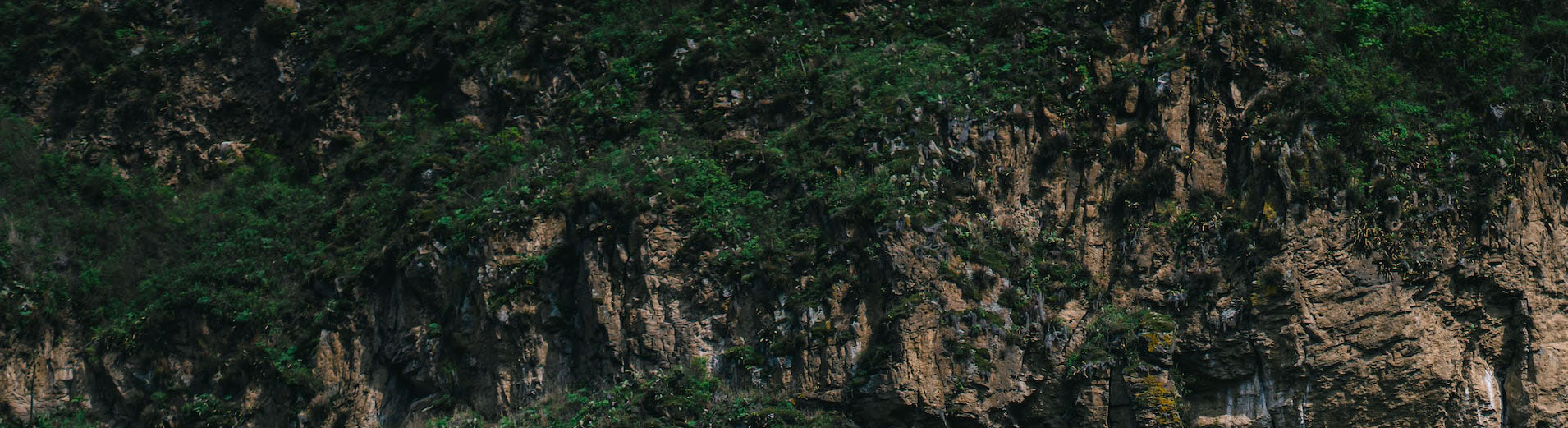
Downloads
Authors
Abstract
The status of kelp forests and their vulnerability to climate change are of global significance. As the foundation for productive and extensive ecosystems, understanding the long-term trends in kelp is critical to coastal ecosystem management, climate resiliency, and restoration programs. In this study, we curate historical US government kelp inventories, develop methods to compare them with contemporary surveys, and use a machine learning framework to evaluate and rank the drivers of change for California kelp forests over the last century. Historical surveys documented Macrocystis and Nereocystis kelp forests covered approximately 120.4 km2 in 1910-1912, which is only slightly above surveys in 2014-2016 (112.0 km2). These statewide comparisons, however, mask dramatic regional changes with increases in Central California (+57.6%, +19.7 km2) and losses along the Northern (-63.0%, -8.1 km2), and Southern (-52.1%, -18.3 km2) mainland coastlines. Random Forest models rank sea otter (Enhydra lutris nereis) population density as the primary driver of kelp changes, with benthic substrate, extreme heat, and high annual variation in primary productivity also significant. This century-scale perspective identifies dramatically different outcomes for California’s kelp forests, providing a blueprint for nature-based solutions that enhance coastal resilience to climate change.
DOI
https://doi.org/10.32942/X2B30R
Subjects
Life Sciences, Physical Sciences and Mathematics
Keywords
Nature-based solutions, climate resiliency, historical ecology, keystone species, Heat Extremes, natural climate solutions
Dates
Published: 2023-08-26 09:11
Last Updated: 2023-11-14 22:41
Older Versions
License
CC BY Attribution 4.0 International
Additional Metadata
Language:
English
Conflict of interest statement:
The authors have no conflicts of interest
Data and Code Availability Statement:
All datasets and code used here are available at the third-party repositories GitHub (https://bit.ly/3pvUkQI) and Open Science Framework (https://osf.io/gsjex/).
Comment #131 Kyle Schuyler Van Houtan @ 2023-11-14 22:26
the final published version of this manuscript including the URL and DOI are at: https://doi.org/10.1371/journal.pclm.0000290