This is a Preprint and has not been peer reviewed. The published version of this Preprint is available: https://doi.org/10.1093/ornithapp/duad056. This is version 4 of this Preprint.
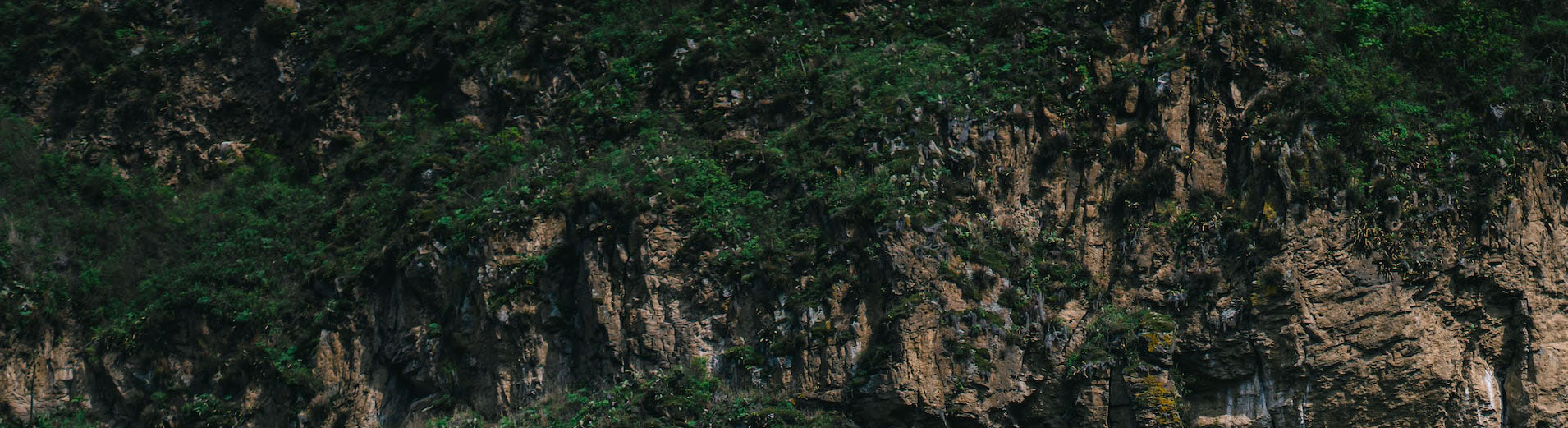
Spatially explicit Bayesian hierarchical models improve avian population status and trends
Downloads
Authors
Abstract
Population trend estimates form the core of avian conservation assessments in North America and indicate important changes in the state of the natural world. The models used to estimate these trends would be more efficient and informative for conservation if they explicitly considered the spatial locations of the monitoring data. We created spatially explicit versions of some standard status and trend models applied to long-term monitoring data for birds across North America. We compared the spatial models to simpler non-spatial versions of the same models, fitting them to simulated data and real data from three broad-scale monitoring programs: the North American Breeding Bird Survey (BBS), the Christmas Bird Count (CBC), and a collection of programs we refer to as Migrating Shorebird Surveys (MSS). All the models generally reproduced the simulated trends and population trajectories when there were many data, and the spatial models performed better when there were fewer data and in locations where the local trends differed from the range-wide means. When fit to real data, the spatial models revealed interesting spatial patterns in trend, such as increases along the Appalachian Mountains for the Eastern Whip-poor-will, that were much less apparent in results from the non-spatial versions. The spatial models also had higher out-of-sample predictive accuracy than the non-spatial models for a selection of species using BBS data. The spatially explicit sharing of information allows fitting the models with much smaller strata, allowing for finer-grained patterns in trends. Spatially informed trends will facilitate more locally relevant conservation, highlight areas of conservation successes and challenges, and help generate and test hypotheses about the spatially dependent drivers of population change.
DOI
https://doi.org/10.32942/X2088D
Subjects
Population Biology
Keywords
abundance, Bayesian, GAM, Hierarchical, iCAR, biological monitoring, spatially explicit
Dates
Published: 2023-05-18 10:50
Last Updated: 2023-12-17 01:28
Older Versions
License
CC BY Attribution 4.0 International
Additional Metadata
Conflict of interest statement:
None
Data and Code Availability Statement:
https://github.com/AdamCSmithCWS/Spatial_Hierarchical_Trend_Models
Language:
English
There are no comments or no comments have been made public for this article.