This is a Preprint and has not been peer reviewed. This is version 1 of this Preprint.
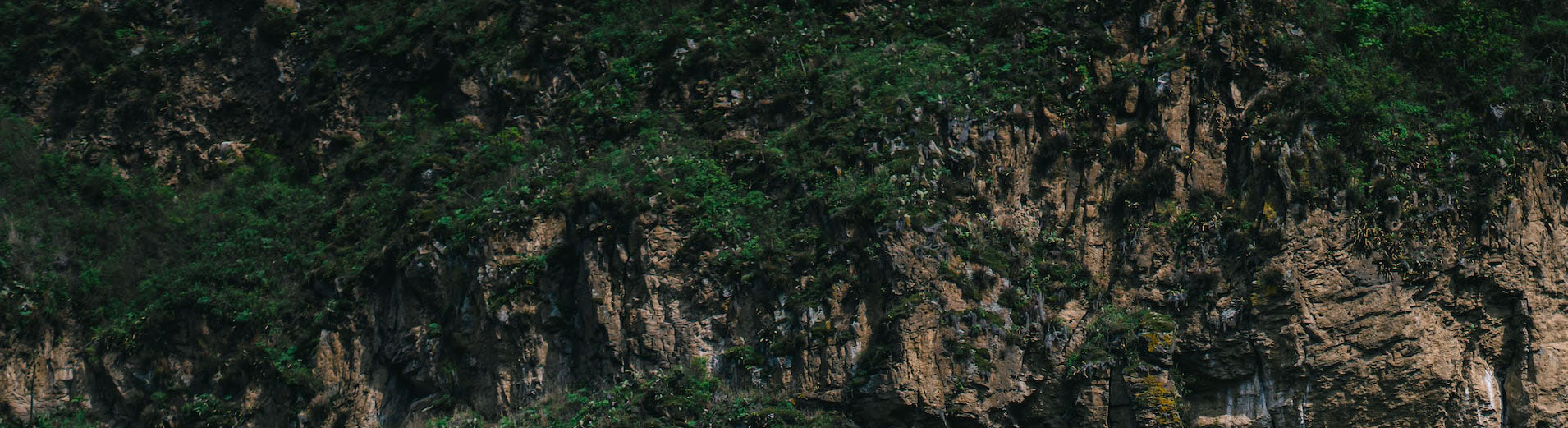
Improving ecological connectivity assessments with transfer learning and function approximation
Downloads
Authors
Abstract
This is a conference paper presented at the ICLR 2023 "Machine Learning for Remote Sensing" workshop.
Protecting and restoring ecological connectivity is essential to climate change adaptation, and necessary if species are to shift their geographic distributions to track their suitable climatic conditions over the coming century. Despite the increasing availability of near real-time and high resolution data for landcover change, current connectivity planning projects are hindered by the computational time required to run connectivity analyses at realistic geographic scales with realistic models of movement. This bottleneck precludes application of optimization algorithms to prioritize ecological restoration to maintain and improve connectivity. Here we propose we can make progress toward overcoming these challenges using machine-learning methods. Our proposed methods will enable rapid optimization of connectivity prioritization and extend its application to many more species than is currently possible. We conclude by illustrating how this project will contribute to efforts to apply connectivity conservation using an example of ongoing restoration in southern Québec.
DOI
https://doi.org/10.32942/X2259C
Subjects
Artificial Intelligence and Robotics, Biodiversity, Environmental Monitoring, Numerical Analysis and Computation, Other Ecology and Evolutionary Biology, Statistical Models, Sustainability
Keywords
connectvity, landscape ecology, machine learning, dispersal, movement, circuitscape
Dates
Published: 2023-05-04 09:20
License
CC-BY Attribution-NonCommercial-ShareAlike 4.0 International
Additional Metadata
Language:
English
Comment #98 Michael D Catchen @ 2023-05-04 14:50
Note: this is a proposal track paper accepted at the Machine Learning for Remote Sensing workshop at ICLR 2023 (https://nasaharvest.github.io/ml-for-remote-sensing/iclr2023/)