This is a Preprint and has not been peer reviewed. This is version 3 of this Preprint.
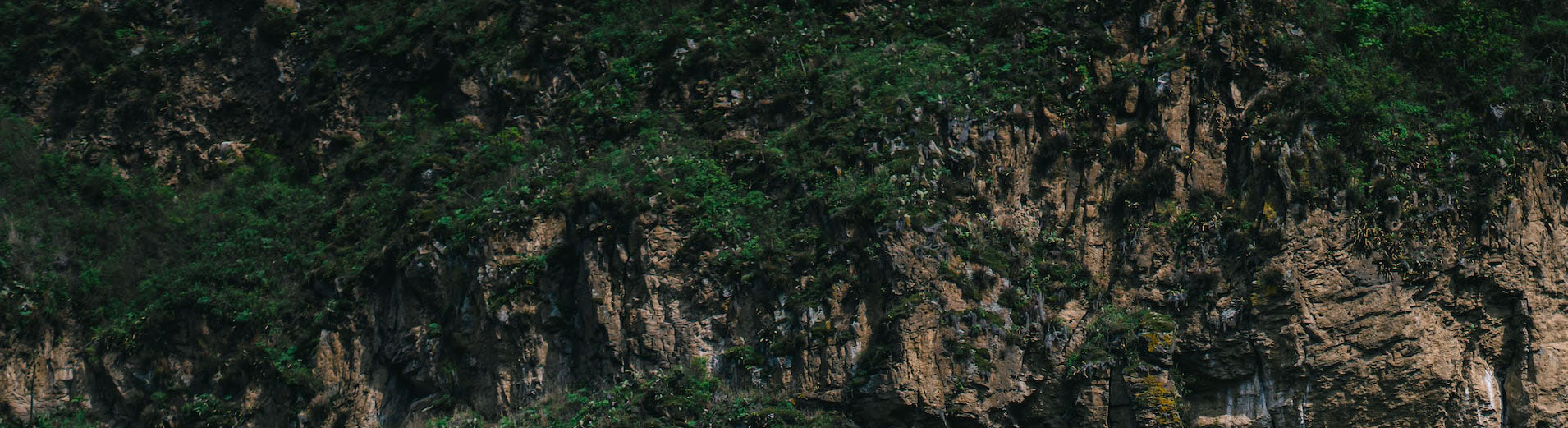
Downloads
Authors
Abstract
Determining the harvest location of timber is crucial to enforcing international regulations designed to protect natural resources and to tackle illegal logging and associated trade in forest products. Stable Isotope Ratio Analysis (SIRA) can be used to verify claims of timber harvest location by matching levels of naturally-occurring stable isotopes within wood tissue to location-specific ratios predicted from reference data (‘isoscapes’). However, overly simple models for predicting isoscapes have so far limited the confidence in derived estimates of timber provenance. In addition, most use cases have limited themselves to differentiating between a small number of pre-determined location options. Here, we present a new SIRA data analysis pipeline, designed to infer the harvest location of a focal tree out of a continuous, arbitrarily large area. We use Gaussian Processes to robustly estimate isoscapes from reference wood samples, and overlay with species distribution data to compute, for every pixel in the study area, the probability of it being the origin of the examined timber. This is the first time, to our knowledge, that this approach is applied to determining timber provenance, providing probabilistic results rather than a binary outcome. Additionally, we include an active learning tool to identify locations from which additional samples would maximize the improvement to model performance, allowing for optimisation of field efforts. We demonstrate our approach on a set of SIRA data from seven oak species in the USA as a proof of concept. Our method can determine the harvest location up to within 520 km from the true origin of the sample and outperforms the state-of-the-art approach. Incorporating species distribution data improves accuracy by up to 36\%. The future-sampling locations proposed by our tool decrease the variance of resultant isoscapes by up to 86\% more than sampling the same number of locations at random. The method we present here greatly advances the toolset available for verification of timber harvest location, will empower authorities worldwide in enforcing anti-deforestation legislation and will help protect natural resources.
DOI
https://doi.org/10.32942/X28304
Subjects
Biodiversity, Biostatistics, Forest Management, Other Forestry and Forest Sciences, Statistical Methodology, Statistical Models
Keywords
SIRA, origin traceability, timber provenance, illegal logging, isoscapes, Gaussian Processes
Dates
Published: 2023-02-23 03:54
Last Updated: 2023-05-30 15:03
Older Versions
License
CC BY Attribution 4.0 International
Additional Metadata
Data and Code Availability Statement:
Code not available (yet)
There are no comments or no comments have been made public for this article.