This is a Preprint and has not been peer reviewed. The published version of this Preprint is available: https://doi.org/10.1111/2041-210X.14209. This is version 3 of this Preprint.
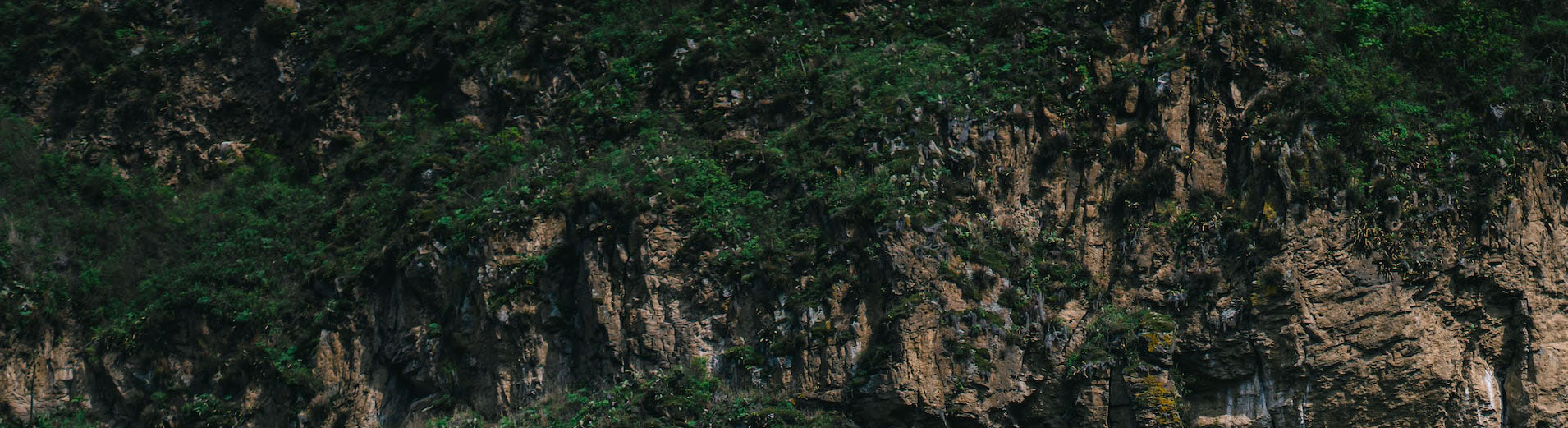
USE it: uniformly sampling pseudo-absences within the environmental space for applications in habitat suitability models
Downloads
Authors
Abstract
1. Habitat suitability models infer the geographical distribution of species using occurrence data and environmental variables. While data on species presence are increasingly accessible, the difficulty to confirm real absences in the field often forces researchers to generate them in silico. To this aim, pseudo-absences are commonly randomly sampled across the study area (i.e., the geographical space). However, this introduces sample location bias (i.e., the sampling is unbalanced towards the most frequent habitats occurring within the geographical space) and favours class overlap (i.e., overlap between environmental conditions associated with species presences and pseudo-absences) in the training dataset.
2. To mitigate this, we propose an alternative methodology (i.e., the uniform approach) that systematically samples pseudo-absences within a portion of the environmental space delimited by a kernel-based filter, which seeks to minimise the number of false-absences included in the training set.
3. We simulated 50 virtual species and modelled their distribution using training datasets assembled with the presence points of the virtual species and pseudo-absences collected using the uniform approach and other approaches that randomly sample pseudo-absences within the geographical space. We compared the predictive performance of habitat suitability models and evaluated the extent of sample location bias and class overlap associated with the different sampling strategies.
4. Results indicated that the uniform approach: (i) effectively reduces sample location bias and class overlap; (ii) provides comparable predictive performance to sampling strategies carried out in the geographic space; and (iii) ensures gathering pseudo-absences adequately representing the environmental conditions available across the study area. We developed a set of R functions in an accompanying R package called USE to disseminate the uniform approach.
DOI
https://doi.org/10.32942/X2XS32
Subjects
Life Sciences
Keywords
reproducibility., reproducibility, species distribution models, class overlap, sample location bias, presence-only models, habitat suitability models, environmental space, ecological niche models, background points, species distribution models, class overlap, sample location bias, presence-only models, habitat suitability models, environmental space, ecological niche models, pseudo-absence
Dates
Published: 2023-02-13 10:02
Last Updated: 2023-06-24 10:08
Older Versions
License
CC BY Attribution 4.0 International
Additional Metadata
Conflict of interest statement:
No conflict of interest has been declared by the authors.
Data and Code Availability Statement:
The scripts for replicating the analyses presented in this paper are available at https://github.com/danddr/USE_paper, as well as all the raw outputs of the simulations and statistical analysis, which are available as an .RDS file. We provide a tutorial to explain how to apply the uniform approach to real case studies, using the European beech, Fagus sylvatica L. as a target species in S4. The R script of the tutorial is available at https://github.com/danddr/USE_paper.
There are no comments or no comments have been made public for this article.