This is a Preprint and has not been peer reviewed. The published version of this Preprint is available: https://doi.org/10.1186/s13750-023-00301-6. This is version 1 of this Preprint.
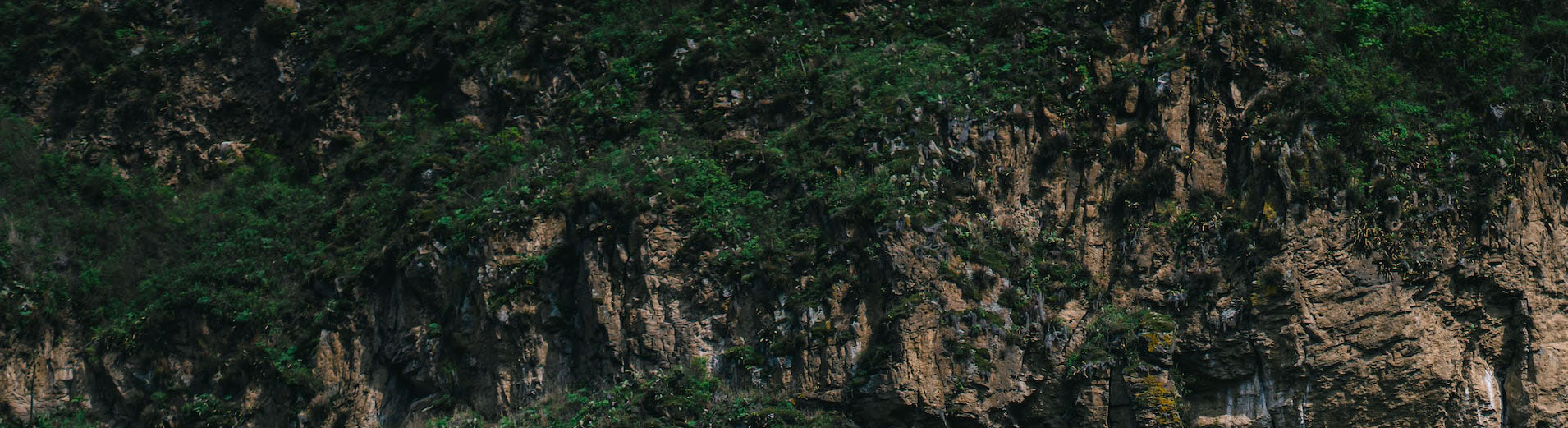
Quantitative evidence synthesis: a practical guide on meta-analysis, meta-regression, and publication bias tests for environmental sciences
Downloads
Supplementary Files
Authors
Abstract
Meta-analysis is a quantitative way of synthesizing results from multiple studies to obtain reliable evidence of an intervention or phenomenon. Indeed, an increasing number of meta-analyses are conducted in environmental sciences, and resulting meta-analytic evidence is often used in environmental policies and decision-making. We conducted a survey of recent meta-analyses in environmental sciences and found poor standards of current meta-analytic practice and reporting. Only ~40% of the 73 reviewed meta-analyses reported heterogeneity (variation among effect sizes beyond sampling error), and publication bias was assessed in less than half. Furthermore, although almost all the meta-analyses had multiple effect sizes originating from the same studies, non-independence among effect sizes was considered in only half of the meta-analyses. To improve the implementation of meta-analysis in environmental sciences, we here outline practical guidance for conducting a meta-analysis in environmental sciences. We describe the key concepts of effect size statistics and meta-analysis, and detail procedures for fitting multilevel meta-analysis and meta-regression models and performing associated publication bias tests. We demonstrate a clear need for environmental scientists to embrace multilevel meta-analytic models, which explicitly model dependence among effect sizes, rather than the commonly used random-effects models. Further, we discuss how reporting and visual presentations of meta-analytic results can be much improved by following reporting guidelines such as PRISMA-EcoEvo (Preferred Reporting Items for Systematic Reviews and Meta-Analyses for Ecology and Evolutionary Biology). This paper, along with the accompanying online tutorial (link), serves as a practical guide on conducting a complete set of meta-analytic procedures (i.e., meta-analysis, heterogeneity quantification, meta-regression, publication bias tests and sensitivity analysis) and also as a gateway to more advanced, yet appropriate, methods.
DOI
https://doi.org/10.32942/X2KK5N
Subjects
Life Sciences
Keywords
Hierarchical Models, robust variance estimation, spatial dependency, variance-covariance matrix, meta-analysis of variance, missing data, network meta-analysis, multivariate meta-analysis
Dates
Published: 2023-01-07 12:15
Last Updated: 2023-01-07 20:14
License
CC-BY Attribution-NonCommercial 4.0 International
Additional Metadata
Conflict of interest statement:
None
Data and Code Availability Statement:
https://github.com/itchyshin/Meta-analysis_tutorial
There are no comments or no comments have been made public for this article.